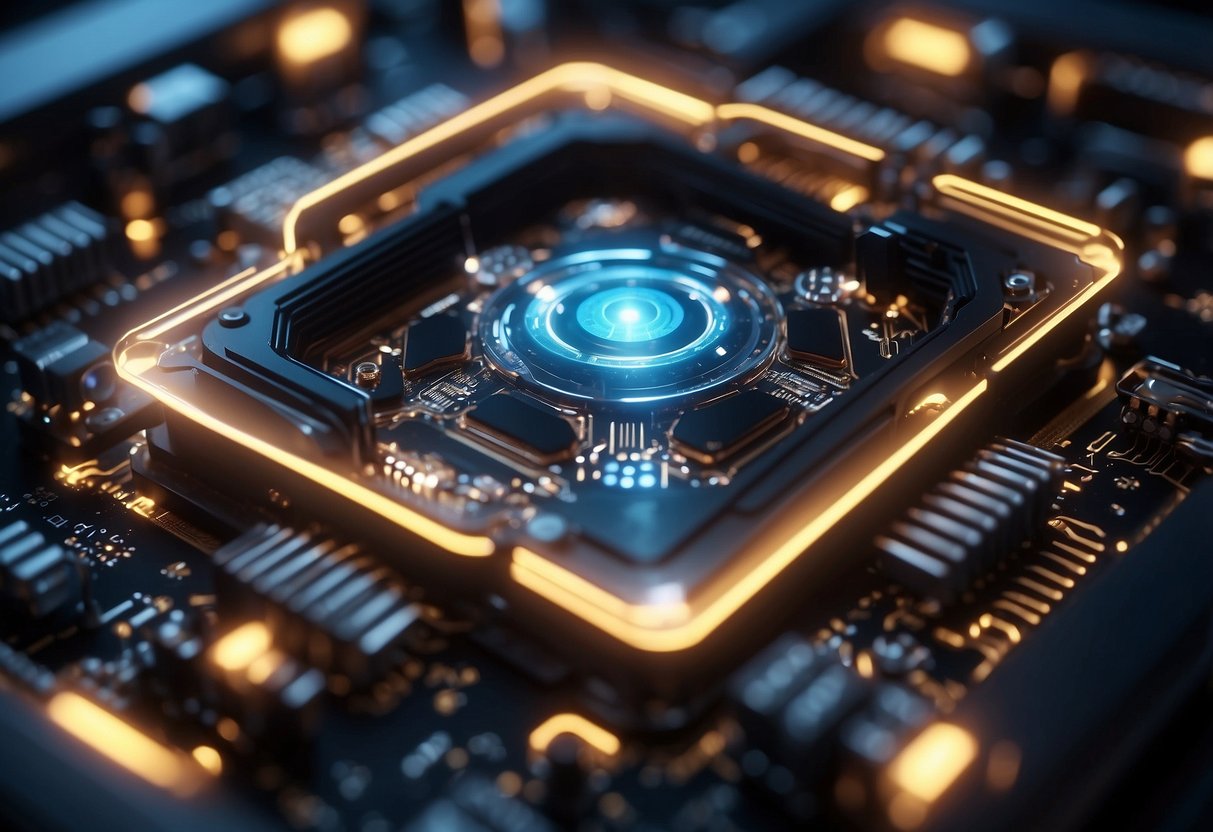
Optimization Problems in Industry
Quantum computing excels in addressing optimization problems, which involve finding the most efficient solution among many possible options. Industries such as logistics, finance, and manufacturing face complex optimization challenges, including route planning, portfolio management, and supply chain optimization. Quantum algorithms can explore numerous possibilities simultaneously, speeding up the process and improving efficiency.
For instance, quantum computers can enhance logistical operations by optimizing delivery routes, reducing fuel consumption, and improving turnaround times. In finance, quantum optimization can lead to better risk management and optimized investment portfolios. Manufacturing processes can also benefit from quantum-enhanced scheduling and resource allocation.
Quantum Computing in Artificial Intelligence
Quantum computing has the potential to revolutionize artificial intelligence (AI) by providing exponential improvements in processing power. Quantum algorithms can handle vast amounts of data more efficiently than classical computers, accelerating machine learning tasks. This enhancement can lead to more accurate predictive models, superior pattern recognition, and faster training times for neural networks.
Quantum machine learning (QML) algorithms are being developed to boost capabilities in natural language processing, image recognition, and decision-making tasks. Integration of quantum computing can also advance AI applications in healthcare, finance, and autonomous systems. The synergy between quantum computing and AI can result in more intelligent systems capable of solving previously intractable problems.
Challenges in Quantum Computing
Quantum computing faces significant hurdles, particularly in technical implementation and material and manufacturing processes. These challenges need addressing to advance the field and achieve practical quantum utility.
Technical Challenges
One of the major technical challenges is error rates. Quantum bits, or qubits, are highly susceptible to errors from environmental interactions, leading to decoherence and loss of quantum information. Implementing error correction methods is crucial but difficult due to the need for numerous additional qubits for redundancy.
Quantum algorithms also face challenges in their development and execution. Designing algorithms that capitalize on quantum speedups is complex, requiring new theoretical advancements and optimization techniques. Additionally, current quantum computers have limited qubit counts, which restricts the size of problems they can handle efficiently.
Another significant hurdle involves maintaining qubit stability. Superconducting qubits, ion traps, and other technologies require extremely low temperatures and precise control, posing substantial engineering challenges. Developing scalable, reliable systems that can operate stably for extended periods is essential but remains a formidable task.
Material and Manufacturing Concerns
Material and manufacturing issues play a critical role in the advancement of quantum computing. Identifying materials that can reliably act as qubits without excessive noise or rapid decoherence is a significant challenge. Materials like superconductors or topological insulators show promise, yet require further investigation to optimize their properties for quantum use.
Manufacturing quantum devices at scale introduces additional complexities. The precision required for fabricating qubits and controlling their interactions necessitates advanced, often custom manufacturing techniques. This precision must be maintained as systems scale up, requiring innovations in nanotechnology and microfabrication.
Furthermore, integrating quantum components with classical computing infrastructure demands seamless interfacing and robust connectivity solutions. This integration is vital for transitioning quantum computing from experimental setups to practical applications, making it a key area of ongoing research and development.