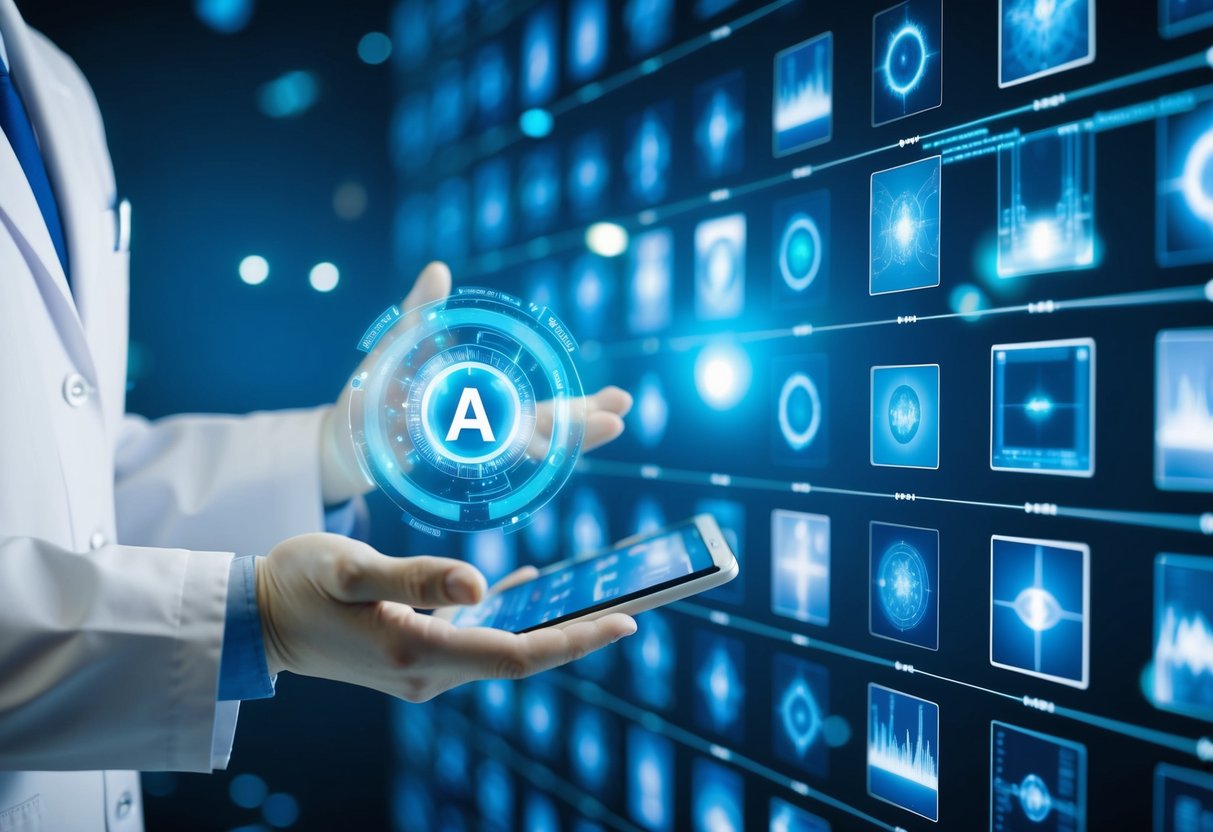
Emerging Technologies and Future Directions in AI-Driven Healthcare
Rapid advancements in AI are reshaping healthcare. Telemedicine, robotics, and deep reinforcement learning are among the key developments at the forefront, each contributing to more efficient, personalized, and accessible medical care.
Innovations in Telemedicine and Remote Diagnosis
Telemedicine has transformed how patients interact with healthcare providers. AI algorithms enhance remote diagnosis by analyzing medical images and patient data, improving accuracy and speed. With wearable technology and mobile apps, patients can monitor chronic conditions from home, facilitating real-time updates to healthcare teams. This integration of AI into telemedicine has led to early detection of diseases, streamlined workflows, and reduced healthcare costs, making quality healthcare accessible regardless of physical location.
The Frontier of AI: Robotics and Virtual Assistants in Clinical Practice
Robotics and virtual health assistants are entering clinical settings, offering support in routine tasks and complex procedures. Surgical robots, powered by AI, allow for minimally invasive surgeries with improved precision. Virtual assistants help manage scheduling, patient inquiries, and medication reminders. These technologies reduce the burden on healthcare providers, improve patient outcomes, and create a more efficient clinical environment by handling routine operations and enabling professionals to focus on more critical tasks.
Deep Reinforcement Learning as the Next Leap in AI Healthcare
Deep reinforcement learning (DRL) is pioneering new possibilities in AI-driven healthcare. By leveraging vast datasets, DRL models develop sophisticated treatment strategies and optimize decision-making processes. These systems can predict patient outcomes, personalize treatment plans, and even simulate complex biological systems. As DRL techniques evolve, they hold the potential to revolutionize disease management and help practitioners make informed decisions based on precise, data-driven insights.
Building an Interdisciplinary Approach to AI Integration
An interdisciplinary approach is essential for effectively integrating AI into healthcare for early detection of chronic diseases. Bringing together expertise from various fields is crucial to harnessing AI’s full potential.
Collaboration Between Clinicians and AI Specialists
Effective AI integration in healthcare demands collaboration between clinicians and AI specialists. Clinicians provide critical insights into patient care, medical protocols, and conditions. AI specialists contribute advanced technical skills to develop and refine algorithms.
Regular interactions between these professionals can lead to innovative solutions that suit clinical settings. Joint training sessions and workshops encourage shared learning and mutual understanding, fostering smoother implementation of AI tools. Open communication helps translate clinical questions into algorithmic solutions, ensuring AI applications align with evidence-based medicine. As a result, clinicians can better trust and utilize AI insights in their day-to-day decision-making processes, improving patient outcomes consistently.
The Importance of Evidence-Based AI Applications
Evidence-based AI applications are a cornerstone of reliable healthcare integration. AI systems should align with the principles of evidence-based medicine to maintain rigor and credibility.
This involves validating AI models with robust datasets from diverse populations, ensuring findings are generalizable and applicable across various clinical scenarios. It is imperative to assess AI tools’ performance against traditional diagnostic methods to verify their efficacy. Collaborators must ensure that AI systems comply with healthcare standards and regulations. Continuous monitoring, evaluation, and refinement of AI systems are vital to adapt to evolving medical knowledge. Engaging in an evidence-based approach also boosts stakeholders’ confidence, supporting broader acceptance and implementation in healthcare environments.