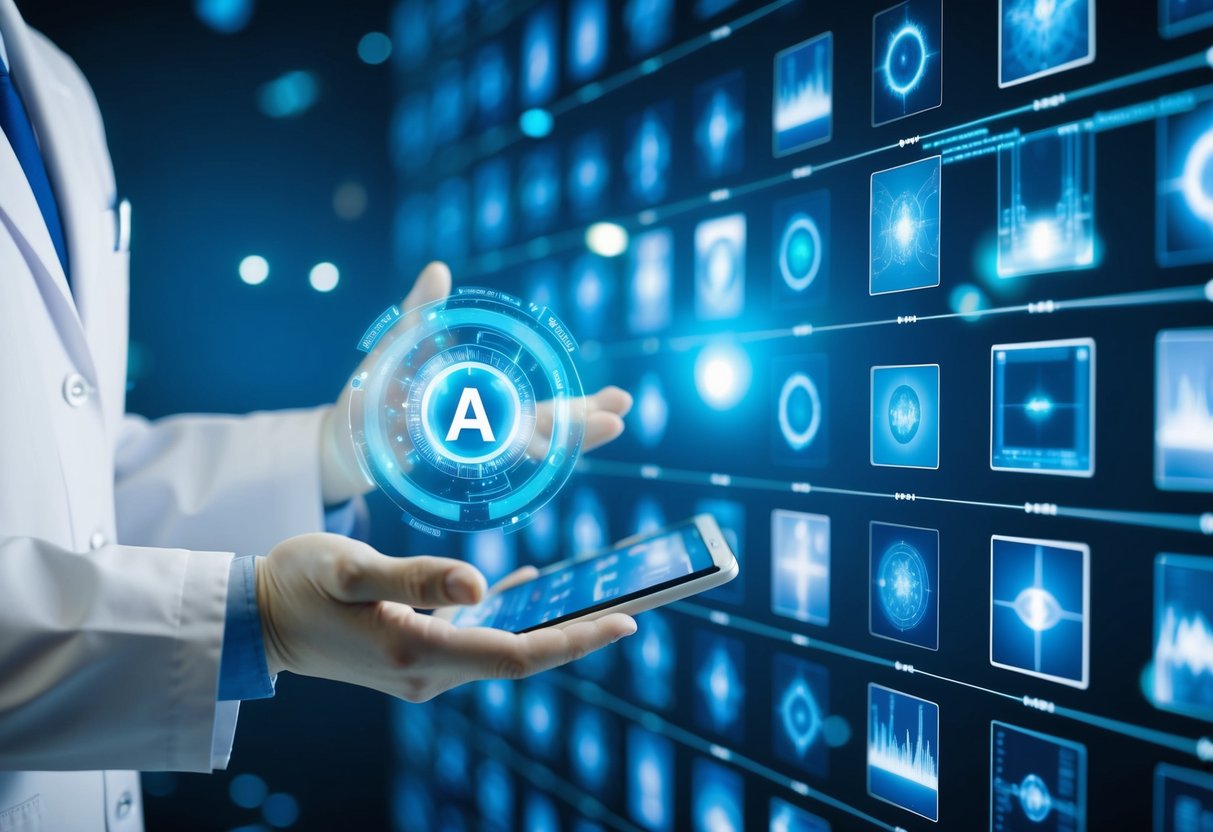
Enhancing Patient Engagement Through AI Tools
AI tools are playing a significant role in increasing patient engagement in their healthcare journey. Personalized health apps offer reminders for medication, exercise, and dietary needs, encouraging adherence to treatment plans. These platforms often include virtual health assistants that provide instant responses to patient queries and support health-related decision-making.
By integrating AI-driven insights with patient preferences and lifestyles, these tools foster a more personalized healthcare experience. The use of chatbots and virtual assistants also enhances communication between patients and healthcare providers, ensuring consistent follow-up and support. This level of engagement not only boosts patient satisfaction but also contributes to better health outcomes by maintaining an ongoing connection between patients and their health management.
Data Analysis and Predictive Modeling in Disease Onset
Accurate data analysis and predictive modeling are crucial in identifying the early stages of chronic diseases. By leveraging advanced algorithms, healthcare professionals can now better understand risk factors and improve clinical outcomes.
Utilizing Predictive Analytics for Risk Assessment
Predictive analytics plays a key role in assessing the likelihood of disease onset. Healthcare data, including medical history and lifestyle factors, is fed into predictive models to generate risk scores. This approach allows for tailored interventions targeting individuals at high risk. Machine learning algorithms learn from vast datasets, identifying patterns that precede chronic disease development. Real-time data processing enhances accuracy, as new information continuously refines predictions. This methodology shifts healthcare from reactive treatment to proactive management, significantly improving patient prognosis.
Bridging Data Analysis with Clinical Decision Making
Integrating data analysis with clinical decision-making enables timely interventions. Clinicians receive insights from predictive models, supporting evidence-based treatment decisions. This synergy between data and practice leads to early diagnosis and personalized care plans. By interpreting analytics results, healthcare providers can recommend lifestyle changes or preventative measures with greater precision. Collaboration between data scientists and clinicians is key to refining model outputs, ensuring they align with practical treatment pathways. This approach enhances patient engagement in their care, reinforcing preventive health strategies and reducing the burden of chronic diseases.
Overcoming Ethical Challenges of AI in Healthcare
Ethical challenges in AI’s integration into healthcare primarily involve patient privacy and decision-making in clinical settings. Balancing innovation with privacy concerns and responsible data use is critical.
Ethical Implications of AI in Clinical Settings
AI’s role in clinical decision-making presents ethical considerations regarding accuracy and bias. Algorithms can influence patient outcomes significantly, thus necessitating transparency in their implementation. Clinicians worry about over-reliance on AI, which could undermine their professional judgment. Ensuring AI technologies adhere to ethical standards is vital for maintaining trust.
AI must complement human expertise without alienating clinicians from decision-making processes. Ethical frameworks should be developed to guide its use in real-world settings. Guiding AI tools to assist in, rather than replace, critical medical decisions helps maintain a balance between innovation and traditional practices.
Responsible Use of AI for Patient Data
AI systems rely heavily on patient data, which raises privacy concerns. Protecting sensitive information requires stringent data handling practices. Data anonymization and secure storage are crucial for preventing unauthorized access and misuse. Patients should be informed about data collection and usage to uphold autonomy and trust.
Regulations such as GDPR in Europe emphasize the importance of consent and transparency. Healthcare institutions should prioritize building frameworks that align with these regulations. Establishing clear guidelines for AI’s data usage will foster a secure environment, ensuring patient data is used ethically and responsibly.