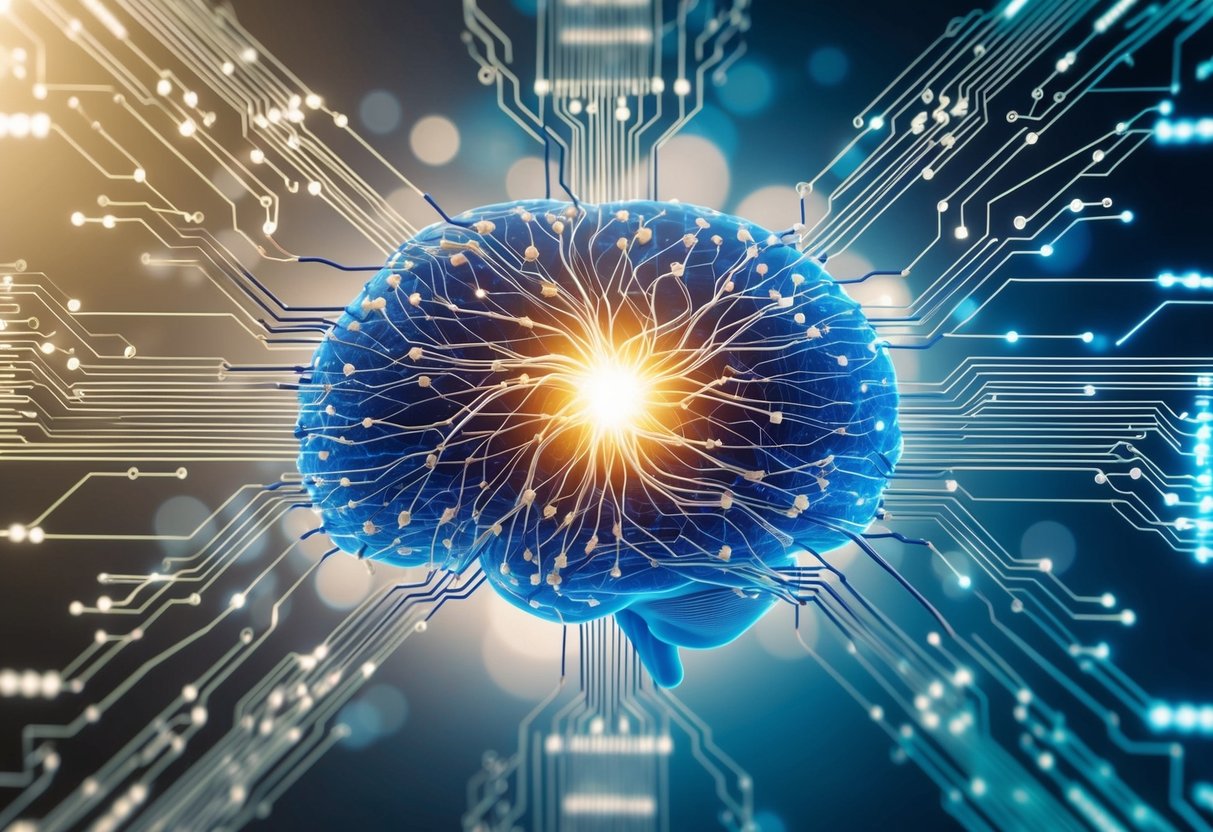
Image and Pattern Recognition
In image and pattern recognition, neuromorphic computing provides a powerful tool for tasks that require quick and accurate data interpretation. Neuromorphic systems excel in recognizing patterns and features from vast sets of visual data, much like a human brain.
The architecture of neuromorphic chips allows for the simultaneous processing of multiple image streams. This leads to enhanced performance in various applications, including surveillance, medical imaging, and facial recognition.
Moreover, these systems are particularly adept at identifying nuances and variations within images. This capability is pivotal for applications in fields like security, where detecting an anomaly swiftly and accurately is critical. Neuromorphic computing further reduces the computational and energy costs typically associated with traditional pattern recognition methods.
Neuromorphic Sensory Systems
Neuromorphic sensory systems aim to replicate the functionality of human senses in devices and applications. By leveraging the strengths of neuromorphic computing, these systems enable devices to process sensory information more effectively.
Such systems are critical in developing more natural and responsive user interfaces. They allow for the perception and interpretation of sensory inputs, such as audio and touch, with heightened sensitivity and minimal latency.
In prosthetics, neuromorphic sensory systems can provide users with a more intuitive and responsive experience. This is achieved by creating patterns of sensory signals that closely mimic those processed by human sensory pathways. By incorporating neuromorphic designs, these technologies enhance the interaction between humans and machines in various innovative applications.
Innovation in AI Technologies
AI technologies continue to evolve, influenced by new approaches like neuromorphic computing. These innovations are focused on enhancing the capabilities of AI systems in areas such as complex problem-solving and real-time decision-making.
From Deep Learning Models to Cognitive Tasks
Deep learning models have significantly advanced machine learning by processing vast amounts of data to identify patterns. Recent developments aim to push AI beyond pattern recognition to enable it to perform cognitive tasks traditionally handled by the human brain. These tasks include reasoning, perception, and language understanding, which require more complex neural networks.
Researchers strive to develop architectures that mimic human cognitive functions. The goal is to enable machines to reason, interpret nuances, and adapt their learning processes effectively. Neuromorphic computing contributes to this shift by offering hardware and frameworks that emulate neurobiological architectures.
Real-Time Processing and Adaptability
The ability of AI systems to process data in real time is crucial for applications that require immediate responses. Neuromorphic computing enhances this capability by providing efficient processing power that mimics synaptic activity. This results in faster data handling and improved adaptability in dynamic environments.
AI systems benefit from increased flexibility, allowing them to adjust to new information quickly and maintain performance stability. Adaptability is vital in environments with continuous data influx, such as autonomous vehicles or real-time monitoring systems.
These innovations aim to create AI systems that are not only capable of quick data processing but can also offer intelligent responses, adapting swiftly to changing situations.
Key Innovations and Leading Technologies
Neuromorphic computing represents a revolutionary shift in technology, closely emulating the processes of the human brain. This area showcases innovations like IBM’s TrueNorth and Intel’s Loihi, as well as various other emerging systems.
IBM TrueNorth and Intel Loihi
IBM’s TrueNorth and Intel’s Loihi are at the forefront of neuromorphic advancements. TrueNorth features a pioneering design built around a network of one million neurons. Its architecture is radically different, incorporating a spiking-neuron integrated circuit that mimics biological neural functioning. TrueNorth is adept at performing complex tasks while consuming minimal power, demonstrating its potential for applications in AI-driven tasks such as pattern recognition.
In contrast, Intel’s Loihi is noted for its emphasis on learning in real-time. With its architecture comprising 130,000 neurons per chip, Loihi facilitates adaptive learning processes. Loihi’s ability to support continuous learning without requiring a traditional software update reflects its unique capability. These chips are designed to tackle challenges like robotic control and energy-efficient computing, illustrating the significant strides in dynamic, energy-aware processing.
Emerging Neuromorphic Computer Systems
The landscape of neuromorphic computing is rapidly evolving with various new systems. Startups and research bodies are driving progress, aiming to further optimize brain-inspired computing. These emerging systems focus on refining synaptic connections at a micro level, pushing the boundaries of current technology.
Projects emphasize integration with existing technologies, enhancing compatibility and performance across platforms. Advances in these systems are promising improved computational speed and efficiency, harboring the potential for significant impacts across industries. From advanced robotics to deep learning frameworks, enhanced neuromorphic systems aim for sustainable integration and expansion of AI capabilities.