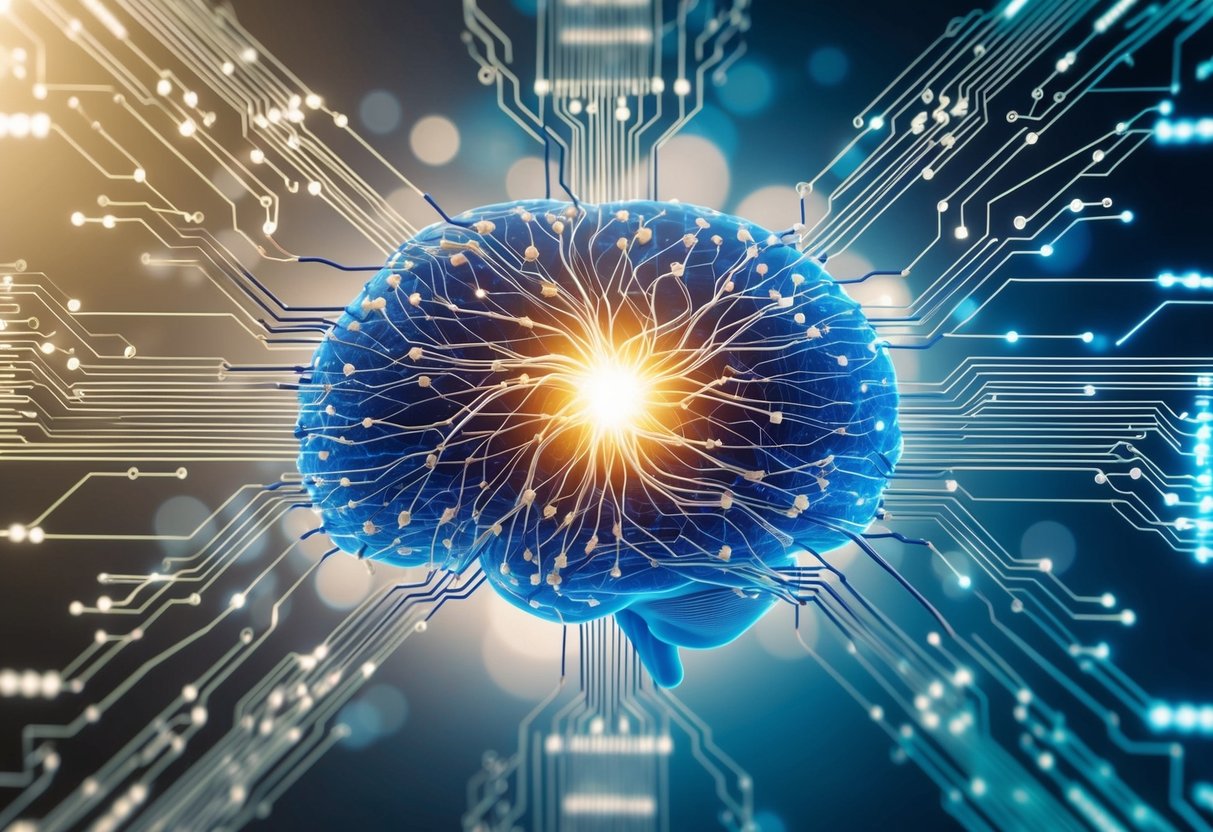
Biological Neural Networks
Biological neural networks consist of interconnected neurons that work together to perform various tasks. Each network contributes to processing distinct types of information, whether sensory, motor, or cognitive. Neural pathways facilitate this communication, allowing for the integration of multiple data inputs.
These networks can reorganize themselves through processes known as neuroplasticity, enabling adaptation and learning. They reflect complex architectures similar to artificial neural networks used in AI, but with a greater degree of flexibility and efficiency. This adaptability underscores the brain’s remarkable ability to recover from injuries and develop new skills. Understanding these networks offers valuable insights into the potential of cognitive computing systems, which aim to replicate such intricate processes.
Cognitive Functions and Processing
Cognitive functions arise from the brain’s capability to process and interpret information. This involves activities such as decision-making, problem-solving, language comprehension, and perception. Different regions of the brain specialize in various tasks, with areas like the frontal lobe playing a pivotal role in reasoning and planning.
Information processing occurs through an intricate blend of electrical impulses and neurotransmitter activities. Cognitive computing seeks to emulate these processes, harnessing the brain’s architecture to create systems capable of intelligent behavior. The aim is to achieve levels of processing efficiency that rival human intelligence, paving the way for advanced AI technologies. This endeavor highlights the importance of understanding the brain’s complex architecture for developing future computing models.
Bridging Biology and Technology
Neuromorphic computing offers a promising path between the realms of biology and technology. By emulating the structure and functionality of the human brain, neuromorphic systems advance artificial intelligence capabilities. These systems focus on replicating brain-like structures and synaptic plasticity to enhance their computational efficiency.
Mimicking Brain-Like Structures
Neuromorphic engineering seeks to replicate the intricate architecture of the human brain, emphasizing spiking neurons. Spiking neurons process information through discrete spikes, rather than continuous signals, mimicking how neurons communicate in biological brains. This approach can potentially reduce energy consumption, offering more efficient computation compared with traditional digital methods.
Neuromorphic systems utilize analog circuits to simulate neural activities, creating models that closely resemble biological neural networks. These systems can recognize patterns, process sensory information, and learn over time, much like the human brain. This imitation of biological features aims to create AI systems capable of performing complex tasks with greater efficiency and adaptability.
Synaptic Plasticity in Neuromorphic Systems
Synaptic plasticity is the cornerstone of learning and memory in biological systems, allowing neural connections to strengthen or weaken over time. Neuromorphic computing incorporates this plasticity into electronic systems to promote adaptability and dynamic learning. By emulating these biological processes, neuromorphic devices can modify their responses based on experience.
In practice, neuromorphic devices adjust synaptic weights through mechanisms inspired by biology. This ability to rewire connections depending on stimuli enables more flexible and intelligent systems. The integration of synaptic plasticity into these systems facilitates real-time learning, promoting advancements in artificial intelligence applications and further bridging the gap between biological processes and technological implementation.