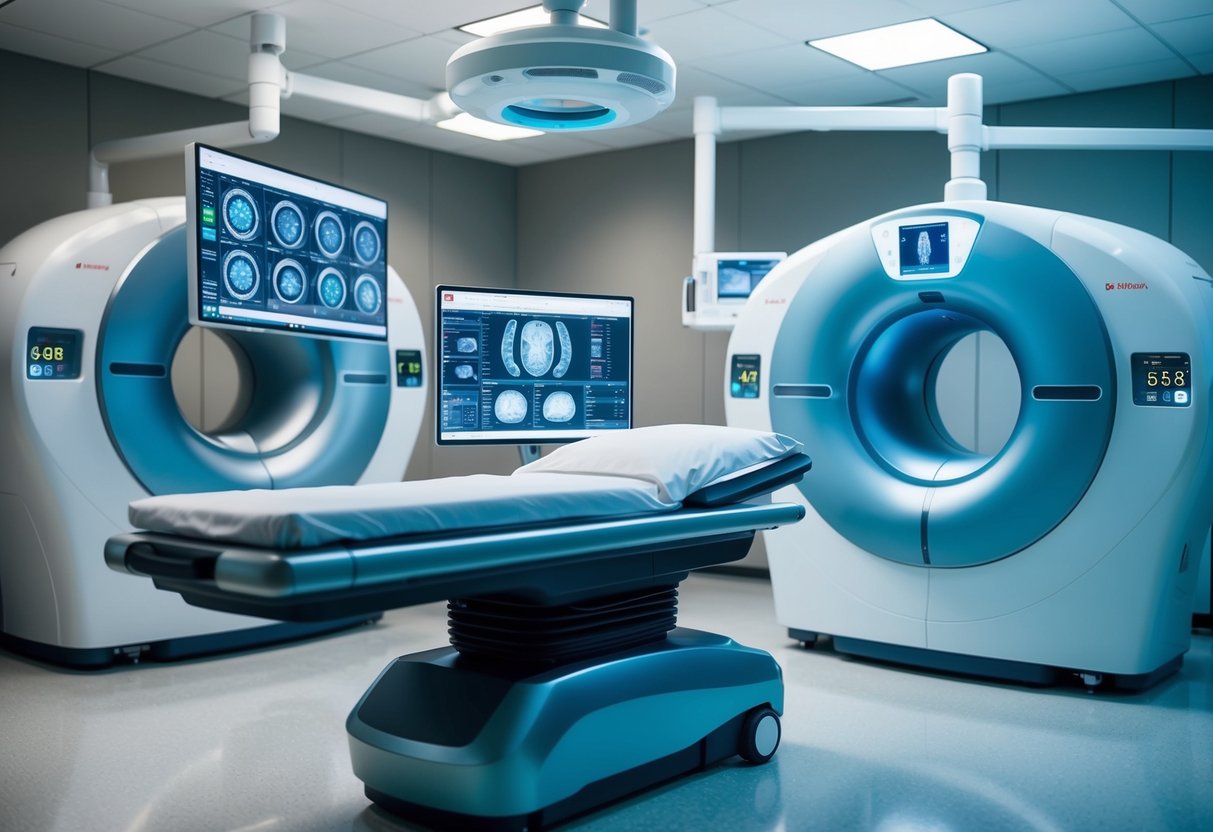
The Role of Big Data in AI Diagnostics
Modern AI diagnostics rely heavily on big data, which serves as the backbone for training and refining AI systems. The effectiveness of these systems is largely contingent upon the quality and breadth of the data they are exposed to.
Datasets and Training for AI Systems
AI systems in the medical field are trained using extensive datasets, which typically include thousands or even millions of medical images. These datasets are crucial for teaching AI systems to accurately identify patterns and anomalies across a wide range of medical conditions.
Training involves feeding these images into the AI model to help it understand what differentiates normal from abnormal findings. Diverse datasets that encompass a wide spectrum of populations, conditions, and imaging techniques enhance the system’s ability to generalize from the data, improving diagnostic accuracy. The availability and variety of big data thus directly influence the model’s performance.
The Significance of Data Quality and Quantity
Data quality is a pivotal factor in the success of AI diagnostics. High-quality datasets ensure that AI systems can make precise and reliable predictions. Issues like missing values, incorrect labeling, or low-resolution images can significantly impair the system’s ability to learn and make accurate diagnoses.
Quantity, too, plays a vital role. Large volumes of data enable AI systems to detect more subtle patterns and gain a deeper insight into complex medical conditions, ultimately refining diagnostic capabilities. Balancing both these elements is essential for developing robust AI systems capable of revolutionizing patient diagnosis.
Ethical and Privacy Considerations
Revolutionizing patient diagnosis with AI brings significant ethical and privacy challenges. Safeguarding patient data and ensuring responsible AI use are crucial considerations that healthcare providers must address.
Data Privacy in Healthcare AI
Healthcare institutions face the challenge of protecting sensitive patient information while leveraging AI technologies. Ensuring data privacy is essential as electronic health records and diagnostic images often contain personally identifiable information.
Implementing protocols like encryption and secure data storage is vital. Robust data anonymization methods help minimize risks of data breaches and unauthorized access. Regulatory frameworks, such as HIPAA in the United States, guide organizations in maintaining compliance and safeguarding patient privacy.
These measures not only protect patients but also build trust between healthcare providers and individuals.
Ethical Concerns Surrounding AI Utilization
Deploying AI in patient diagnosis involves significant ethical considerations. A primary concern is the potential for algorithmic bias, where AI systems may inadvertently perpetuate or amplify existing biases in healthcare data. Ensuring fairness and accuracy requires extensive testing and validation of AI models.
Another critical aspect is patient autonomy. Patients should be informed about AI’s role in their diagnosis and have a say in how their data is used. Transparency in AI decision-making processes is essential to empower patients and enhance their understanding of their healthcare journey.
Balancing innovation with ethical responsibility is crucial to advancing AI in a manner that benefits patients and society.