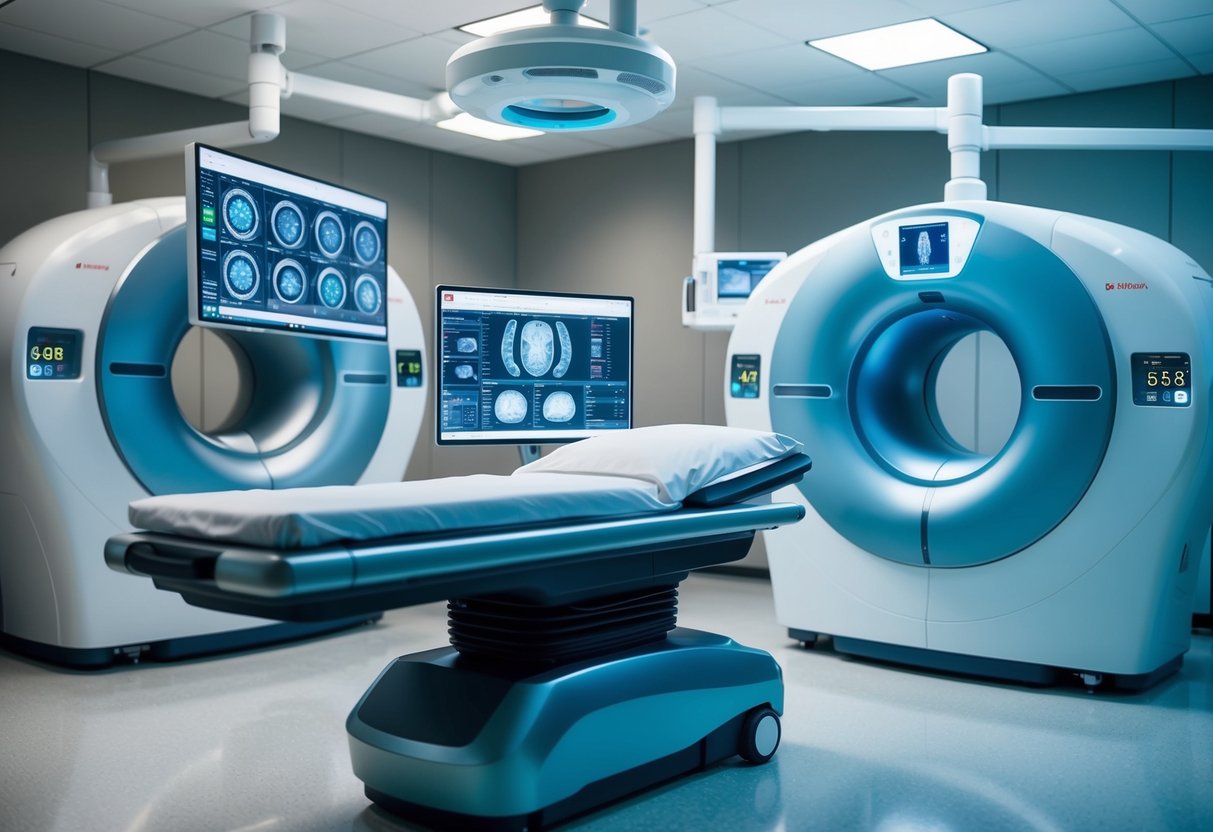
Machine Learning Algorithms and Their Application
Machine learning is transforming medical imaging through its capability to classify and segment images with precision. This section explores deep learning models that are innovating image analysis and diagnosis.
Deep Learning in Image Analysis
Deep learning algorithms have become vital in medical image analysis, particularly for their ability to handle large datasets with numerous features. These models, trained on vast amounts of imaging data, excel in tasks such as tumor detection and anomaly analysis. Their efficiency in identifying subtle patterns invisible to the human eye enhances diagnostic accuracy.
Medical applications commonly use complex models like artificial neural networks, which can process and learn from high-dimensional data. As neural networks improve, their efficacy in image recognition continues to grow. This leads to quicker and more accurate diagnoses, ultimately improving patient outcomes.
Convolutional Neural Networks for Diagnosis
Convolutional Neural Networks (CNNs) play a crucial role in medical diagnosis by breaking down images into individual components for analysis. These networks are especially adept at classification tasks, distinguishing between healthy and diseased tissues. With layers designed to recognize patterns such as edges, corners, and textures, CNNs are effective and reliable tools in radiology and pathology.
CNNs also contribute to image segmentation by accurately identifying organ or tumor boundaries within medical scans. This precision assists radiologists by providing detailed views of the affected areas. Furthermore, CNNs’ application reduces manual workload, allowing healthcare professionals to focus on more complex decision-making tasks in diagnostic processes.
Improving Diagnostic Accuracy with AI
Artificial Intelligence is transforming healthcare by enhancing diagnostic precision. Key developments include early disease detection and reducing errors in imaging interpretation, boosting medical effectiveness.
Early Detection and Disease Classification
AI algorithms now process medical images to identify early signs of diseases. This capability is crucial for conditions like cancer, where early detection significantly affects treatment outcomes. Machine learning models analyze patterns in imaging data, classifying diseases more accurately and swiftly than traditional methods.
Custom-built AI models are adept at identifying subtle changes that might be missed by human eyes. It aids healthcare providers in making well-informed decisions, ensuring faster and more precise diagnosis. This innovation not only saves time but also improves patient survival rates through timely interventions.
Reducing Errors in Imaging Interpretation
Errors in imaging, arising from various human and technical factors, can mislead diagnoses. AI helps minimize these errors by evaluating images with exceptional consistency and accuracy. It offers a reliable second opinion, reinforcing diagnostic confidence among healthcare professionals.
Through this technology, complex imaging tasks become more manageable, allowing radiologists to focus on cases requiring human insight. The integration of AI in this context stands to enhance overall diagnostic reliability, reducing false positives and negatives. Thus, AI plays an indispensable role in advancing medical imaging interpretation efficiency.