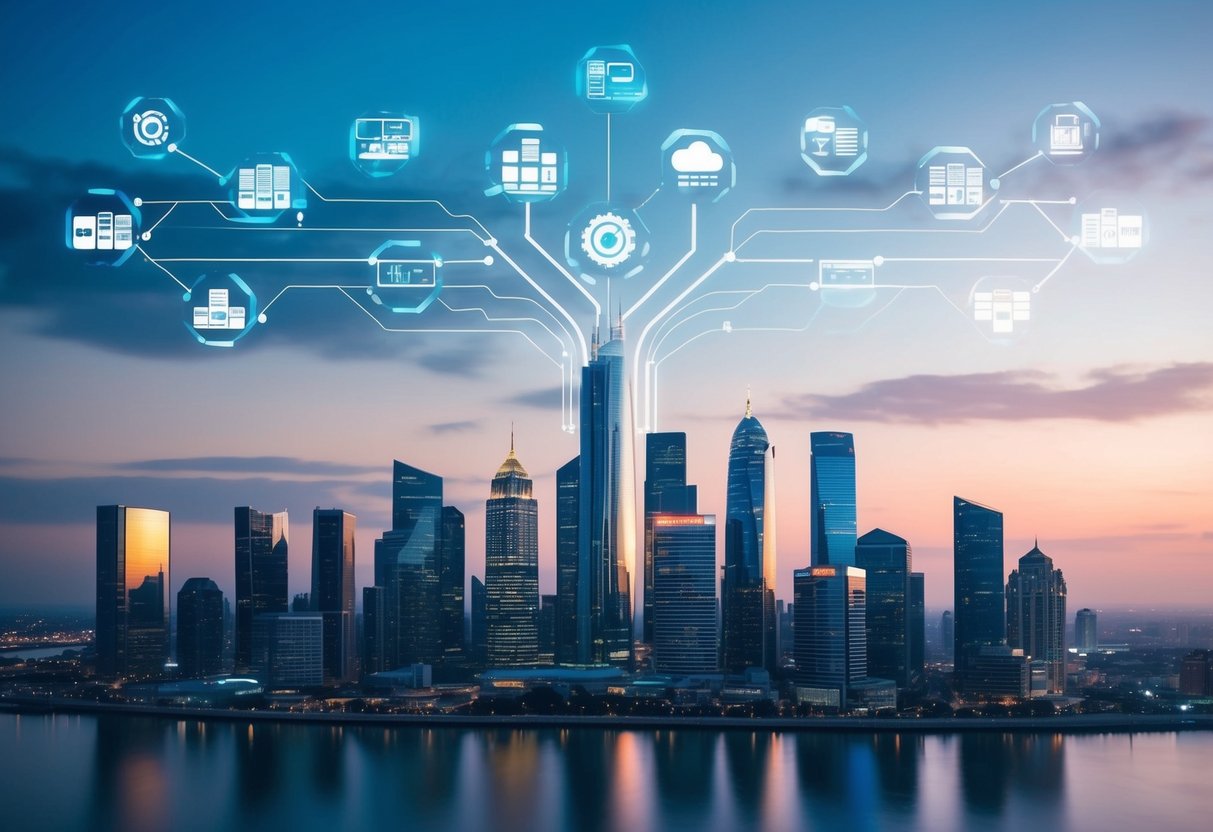
Customer Service and Churn Prediction
In customer service, AI empowers businesses to offer more personalized interactions. Chatbots and virtual assistants use past customer interactions to provide relevant solutions quickly. By understanding individual needs, AI can elevate the customer experience, ensuring clients receive the assistance they require efficiently.
Churn prediction plays a critical role in retaining customers. AI systems analyze data such as customer behavior and transaction history to identify those at risk of leaving. By taking proactive measures, businesses can address issues before they lead to customer loss. Personalized outreach and interventions demonstrate to customers that their satisfaction is a top priority, thus reducing churn rates and maintaining a strong customer base.
Scalability and Future of AI in Business
Scalability and strategic decision-making are critical components in utilizing AI for business growth. Companies can leverage predictive analytics to enhance their decision-making processes and adapt to future trends with precision.
Scaling Predictive Analytics for Growth
Businesses must ensure that predictive analytics systems are scalable to accommodate growth demands. This involves implementing flexible data infrastructures that can handle increased data volumes efficiently. Companies should invest in adaptable AI models that consistently improve with new data inputs, offering precise insights.
Scalability enables firms to extend predictive capabilities across various departments, supporting unified growth. Through scalable systems, businesses can efficiently respond to forecast trends and evolving market dynamics.
Predictive Analytics and Strategic Decision Making
Predictive analytics plays a pivotal role in strategic decision-making by providing actionable insights. It helps businesses anticipate market shifts, customer preferences, and potential risks, enabling informed decisions. By analyzing historical data, companies can predict future trends with higher accuracy.
This foresight facilitates strategic planning, enhancing competitive advantage. Organizations that incorporate predictive analytics into decision-making processes often experience more robust performance and adaptive strategies. This continuous improvement cycle fosters a proactive business environment, ready to address emerging challenges.
Challenges and Ethical Considerations
Predictive analytics in AI holds transformative potential for business decision-making but faces certain challenges and ethical dilemmas. Considerations such as accuracy and ethical usage are key to leveraging this technology effectively.
Overcoming Predictive Analytics Challenges
One major challenge is ensuring the accuracy of predictive models. The quality of data is crucial; inaccurate or incomplete data can lead to misguided insights. Businesses must invest in robust data collection and cleaning processes. They should also continually evaluate model performance to maintain high standards.
Resource allocation is another hurdle. Implementing predictive analytics systems demands significant time and financial resources. From acquiring advanced technologies to hiring skilled data scientists, companies need to allocate resources wisely to reap the benefits of predictive analytics.
Ethics of Predictive Analytics in AI
Ethical concerns in predictive analytics primarily revolve around issues like bias and privacy. AI systems can inadvertently perpetuate biases if trained on skewed datasets. This can lead to unequal treatment and unfair outcomes in areas like recruitment or credit approval. Ethics thus necessitates careful dataset selection and ongoing model assessments to mitigate bias.
Privacy is another critical ethical concern. Predictive analytics often requires vast amounts of personal data, raising questions about consent and data protection. Organizations must be transparent about their data practices and ensure compliance with relevant privacy laws to build trust with stakeholders and maintain ethical standards in their AI applications.