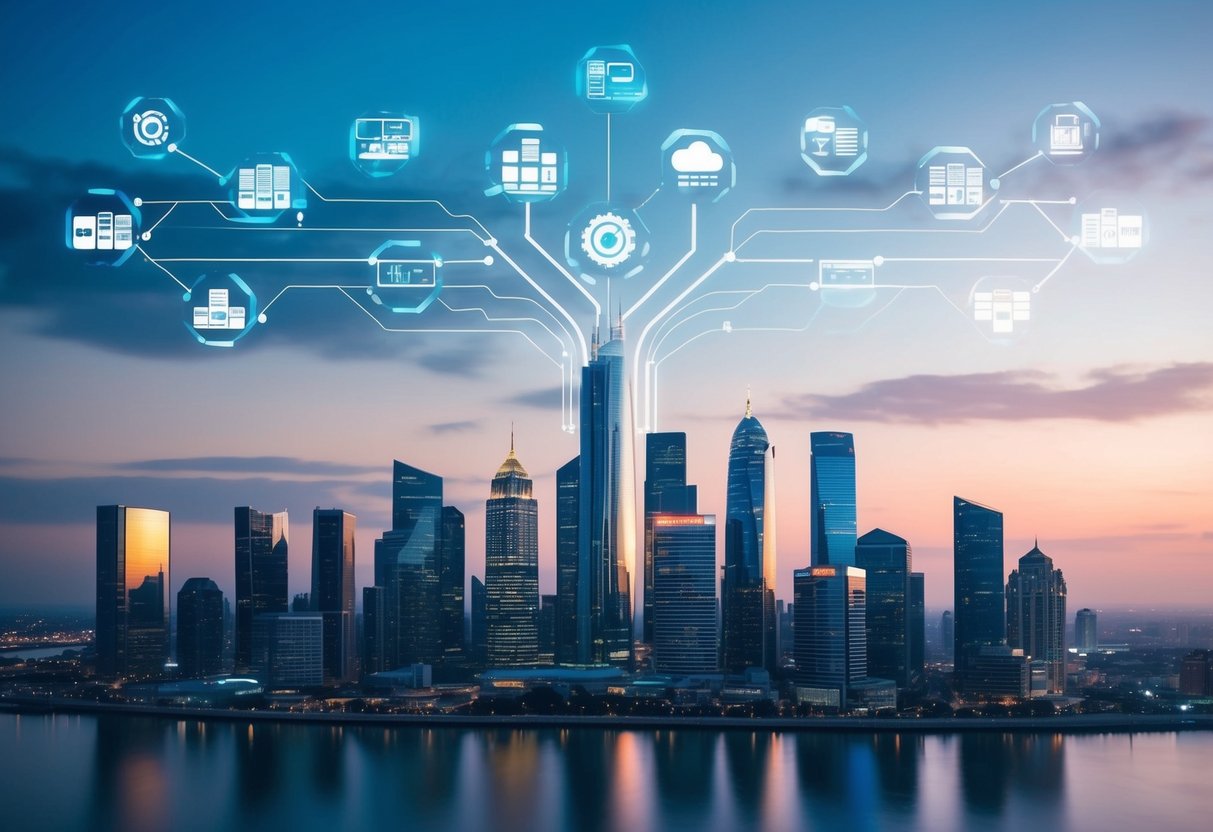
Applications of Predictive Analytics in Various Industries
Predictive analytics plays a vital role across multiple sectors, enhancing efficiency, forecasting trends, and improving overall strategic decisions. Its impact on industries such as healthcare, retail, and manufacturing is transformative, offering solutions that streamline operations and meet consumer demands effectively.
Healthcare Sector
In the healthcare industry, predictive analytics is crucial for patient care and management. It assists in predicting disease outbreaks and personalizing patient treatment plans. Hospitals use predictive models to manage patient admissions by forecasting bed occupancy rates.
This technology is valuable for managing chronic diseases by analyzing patient data to anticipate health deteriorations. Insurers also benefit, identifying high-risk patients to devise targeted interventions, potentially reducing long-term costs. Furthermore, predictive analytics aids in drug discovery, helping expedite the development of new therapies by analyzing biological and clinical data.
Retail and E-Commerce
Retail and e-commerce industries leverage predictive analytics to enhance customer experiences and optimize inventory management. It is used to forecast demand, ensuring stock levels align with consumer needs and reducing overstocking or stockouts.
Customer behavior analysis enables retailers to offer personalized marketing, driving customer engagement and sales. By evaluating purchase patterns and preferences, retailers can tailor promotions effectively. Furthermore, predictive analytics helps in dynamic pricing, adjusting prices in real-time based on demand fluctuations and market conditions. These strategies, in turn, enhance revenue and operational efficiency.
Manufacturing and Supply Chain
In manufacturing and supply chain management, predictive analytics is pivotal in improving operational efficiency. Predictive maintenance minimizes machine downtime by foreseeing potential failures, allowing for timely repairs and resource savings.
This approach aids demand forecasting, improving production scheduling and aligning supply chain activities with market demand, thereby minimizing waste and surpluses. Moreover, logistics benefit from route optimization, reducing transportation costs and delivery times. By analyzing historical data and external factors, manufacturers and supply chain managers can enhance decision-making processes, ensuring better resource allocation and improved customer satisfaction.
Enhancing Performance and Efficiency
Predictive analytics in AI empowers businesses to improve operational processes, manage risks, and ensure high-quality outcomes. By implementing advanced solutions, companies can achieve streamlined operations and proactive measures to address issues before they arise.
Boosting Operational Efficiency
By leveraging AI-powered predictive analytics, organizations can significantly enhance their operational efficiency. Machine learning algorithms process vast amounts of data to forecast trends, demand, and potential bottlenecks. This capability allows businesses to optimize inventory levels, reduce waste, and allocate resources effectively.
For instance, predictive models can anticipate equipment maintenance needs, thereby minimizing downtime and extending machinery life. This AI-driven foresight assists in scheduling maintenance activities efficiently, ensuring uninterrupted production cycles. Moreover, AI applications can identify patterns in consumer behavior, assisting companies in tailoring their strategies to meet real-time market demands, which ultimately boosts productivity and customer satisfaction.
Quality Control and Risk Management
Predictive analytics plays a crucial role in quality control by identifying defects and abnormalities in products before they reach the consumer. By analyzing historical production data, AI systems can determine indicators of potential quality issues. This proactive approach enhances product reliability and reduces costs associated with recalls or customer complaints.
In risk management, AI tools assess potential risks by evaluating internal and external data sources. For example, in supply chain management, predictive analytics can forecast disruptions due to natural disasters or supplier failures. This insight allows for strategic planning and the implementation of contingency measures. These predictive capabilities equip organizations to safeguard their operations against unforeseen challenges, ensuring sustained performance and stability.