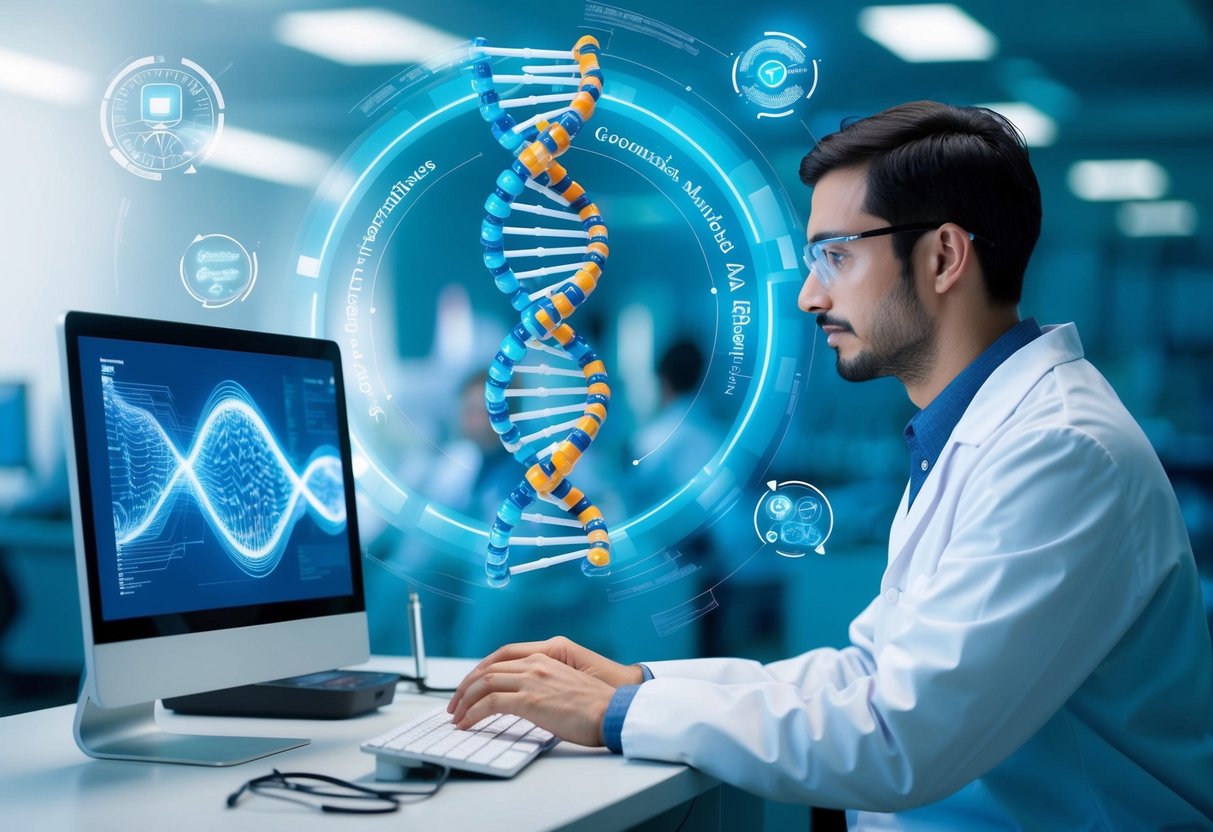
Challenges and Future Directions in AI-Enhanced Genomics
AI-enhanced genomics holds promise for personalized medicine but also faces significant challenges. Key issues include the integration of diverse datasets and the adaptation of research findings into clinical settings.
Interoperability and Data Integration
One challenge in AI-enhanced genomics is the seamless integration of diverse datasets. Genomic data often come from multiple sources, including sequencing data, clinical records, and research databases. This diversity makes interoperability a complicated task. Effective data integration demands standardized data formats and comprehensive frameworks to ensure that information from multiple sources aligns correctly.
Interdisciplinary collaboration is essential to tackle these challenges. Computer scientists, geneticists, and clinicians must work together to develop algorithms and platforms that facilitate data sharing across disciplines. Efforts are also needed to maintain data privacy and security without impeding research advancements. Enhanced cooperation can lead to innovative solutions that improve the utility of data collected and analyzed in genomics research.
Translational Research and Clinical Trials
Another area of focus is the translation of AI-based genomic research into clinical settings. Translational research aims to bridge the gap between laboratory findings and real-world applications. Currently, many algorithms show potential but remain confined to research labs, partly due to regulatory hurdles and lack of clinical validation.
Clinical trials play a vital role in this transition, offering a framework to test AI applications in healthcare settings. These trials require robust methodologies to assess efficacy and safety, ensuring that AI tools are reliable for patient care. Interdisciplinary collaboration is again crucial, as collaboration can streamline the trial process by leveraging expertise across various fields, helping bridge the gap between research and clinical application.
Patient-Centered Approaches to Genomic Medicine
Integrating genomics into patient care holds the promise of creating more personalized treatment plans. This involves carefully considering patient outcomes and utilizing electronic health records to tailor medical interventions for specific patient populations.
Incorporating Patient Outcomes in Treatment Plans
Patient outcomes play a crucial role in the development of personalized treatment plans. By analyzing how patients respond to different genomic interventions, healthcare providers can refine treatments to better suit individual needs. This approach requires a comprehensive understanding of the patient’s genetic makeup and how it interacts with various treatments.
Leveraging this knowledge enables healthcare professionals to design plans that improve patient well-being. Monitoring outcomes allows for adjustments and modifications, ensuring that the benefits of genomic medicine are maximized. By prioritizing outcomes, healthcare systems can also address the unique requirements of different patient populations, enhancing care quality and effectiveness.
The Role of Electronic Health Records
Electronic Health Records (EHRs) are essential tools in genomic medicine, offering a robust platform for storing and analyzing patient data. They support the integration of genetic information with other health metrics, facilitating more targeted and effective treatment strategies. EHRs make it easier to track patients’ progress and tailor treatment adjustments based on real-time data.
The interoperability of EHRs ensures that healthcare providers have access to a comprehensive view of a patient’s health history, enabling informed decision-making. This efficient data management allows for seamless coordination among multiple healthcare providers, enhancing the implementation of personalized treatments across diverse patient populations.