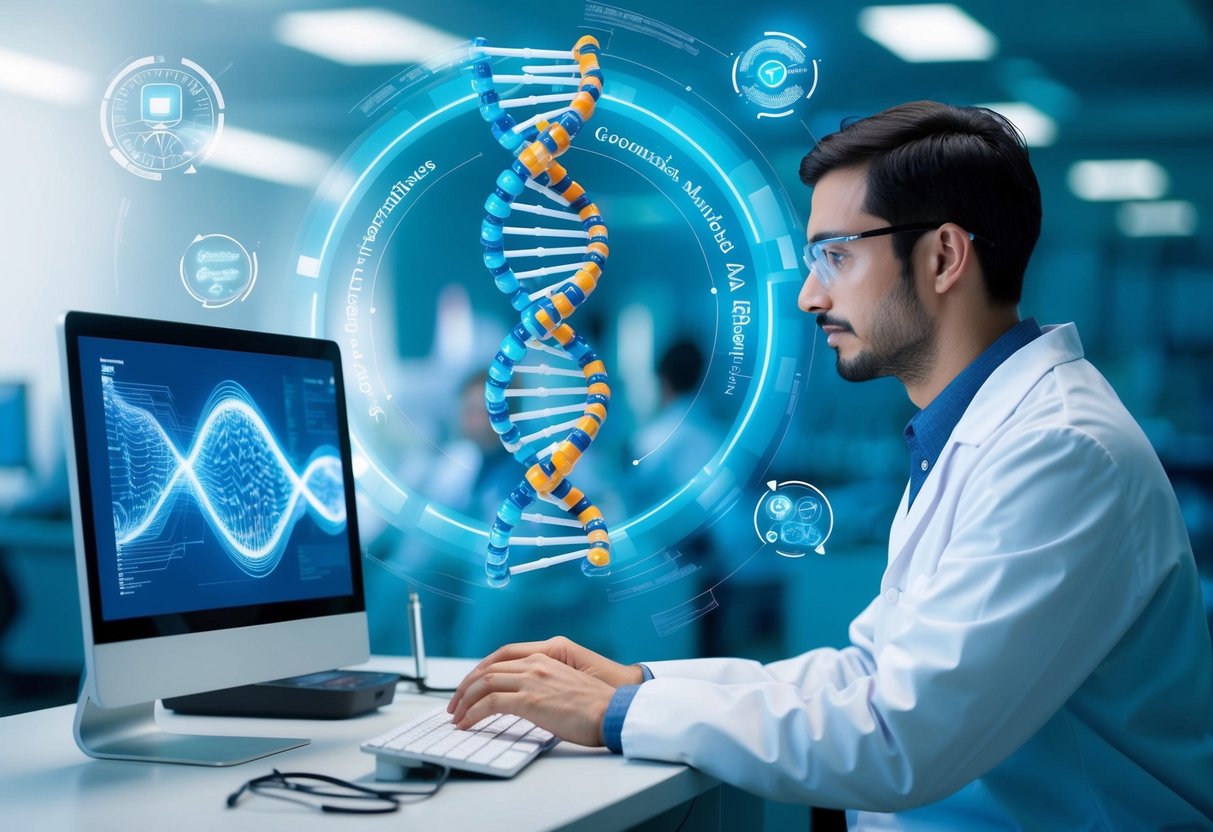
Predictive Models for Genetic Conditions
Predictive models developed through AI are pivotal for identifying individuals at higher risk of genetic conditions. Machine learning algorithms analyze both genomic and phenotypic data to forecast disease predispositions. This enables physicians to tailor preventive measures and therapies for at-risk individuals, personalizing healthcare approaches effectively.
These models also reduce costs by potentially minimizing the need for extensive diagnostic tests and facilitating early interventions. The integration of AI in predictive modeling streamlines the process of customizing treatments based on genetic profiles, thus enhancing patient outcomes. Consequently, AI-driven predictive models represent a significant advancement in the endeavor to offer personalized medicine solutions.
Precision Oncology and AI Integration
Precision oncology leverages AI to enhance cancer diagnosis and tailor treatments based on individual patient profiles. Machine learning algorithms analyze vast data sets to identify nuanced patterns, transforming the landscape of cancer care.
AI’s Transformation of Cancer Diagnosis
AI applications are revolutionizing how cancer is diagnosed by utilizing machine learning to analyze imaging data and genomic information. Tools now outperform traditional methods in detecting anomalies in medical images like X-rays and MRIs. For example, AI systems trained on the Cancer Genome Atlas can identify genetic alterations that suggest disease presence. This leads to earlier and more accurate diagnostics, aiding clinicians in devising precise treatment plans. Algorithms also assist in differentiating between benign and malignant tumors, reducing unnecessary biopsies and interventions.
Tailored Treatments for Oncology Patients
Personalized treatment plans have become achievable through AI’s predictive capabilities. By integrating genomic data, AI can forecast how a patient will respond to various therapies, leading to more effective treatment regimens. In oncology, AI models can analyze patient-specific cancer mutations and predict chemotherapy effectiveness, offering more personalized solutions. These models consider unique genetic profiles, ensuring patients receive highly targeted therapies. This level of specificity reduces the trial-and-error approach traditionally seen in cancer treatment, minimizing side effects and improving patient outcomes.
Ethical and Privacy Perspectives in Genomic AI
The integration of AI and genomics presents opportunities for personalized medicine but raises significant ethical and privacy concerns. Balancing the benefits of technological advancement with the need to protect sensitive genetic data is crucial.
Data Privacy and Security Challenges
Incorporating AI into genomics involves managing large genetic datasets. These datasets contain highly sensitive information, posing a risk if improperly handled. Protecting genetic data from unauthorized access and misuse is a major challenge. Secure storage and data encryption are essential to prevent breaches.
Legal frameworks, like the GDPR, emphasize user consent and data protection, but compliance is complex. Organizations must ensure data is anonymized to mitigate privacy risks. Developing advanced encryption methods and access controls is vital. Implementing robust cybersecurity measures prevents potential exploitation.
Ethical Considerations in Genomics AI
Ethical issues in genomic AI revolve around consent, data ownership, and potential misuse. Individuals must be informed about how their genetic data will be used. There is often uncertainty over data ownership; individuals may not have control once data is shared.
The potential for discrimination based on genetic information is a significant ethical concern. Insurance companies or employers might misuse genomic data, leading to bias. Bioethicists argue for transparent policies, ensuring equitable access to genomic advancements. Ensuring AI systems are unbiased and fair in their analysis is critical to addressing these ethical dilemmas.