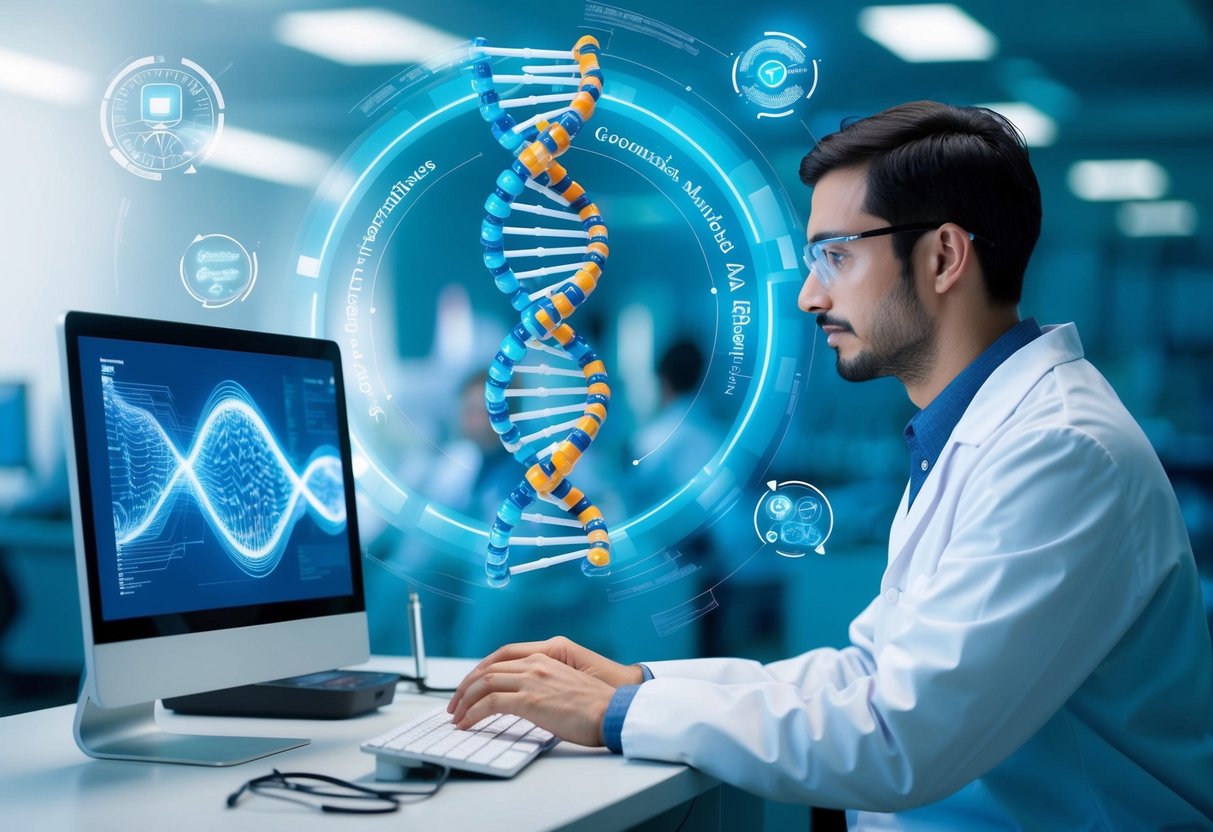
AI’s Role in Healthcare Innovation
AI’s influence in healthcare can be seen through improved diagnostic accuracy and treatment customization. Machine learning algorithms analyze complex datasets, including genomics and patient histories, to identify potential health risks. These innovations aid in developing new therapeutic strategies and managing chronic conditions. AI systems also support medical professionals by automating routine tasks, allowing more focus on patient care. Adoption of these technologies increases efficiency, reduces costs, and enhances the overall quality of healthcare delivery.
AI-Driven Drug Discovery and Development
AI technologies are revolutionizing the drug discovery and development landscape. They enable the analysis of vast datasets, leading to the identification of promising drug candidates and predicting their potential efficacy.
Accelerating Drug Development with AI
AI algorithms play a crucial role in accelerating the drug development process. By automating labor-intensive steps, AI significantly reduces the time needed to identify viable drug candidates. This enables researchers to handle large chemical databases swiftly and efficiently.
AI-driven tools often analyze molecular interactions and properties, rapidly narrowing down thousands of compounds to a few promising candidates. These algorithms are particularly useful for identifying novel drug interactions that could be overlooked with traditional methods.
Incorporating AI into drug development also reduces the cost associated with laboratory experiments. Machine learning models predict outcomes based on existing data, minimizing unnecessary experimental trials. This streamlining benefits pharmaceutical companies by lowering their expenditure and shortening drug development timelines.
Machine Learning for Drug Efficacy Prediction
A key advantage of machine learning in drug discovery is its capability to predict drug efficacy. These models are trained on historical clinical trial data to identify patterns that indicate successful treatments. By understanding how certain molecules act within the body, machine learning models forecast the potential success of new drug candidates.
Predictive modeling is particularly useful in personalized medicine, where treatments are tailored to individual genomic profiles. Machine learning analyzes genetic data to predict how specific drugs will perform for different genetic makeups, enabling the development of more effective treatments.
Additionally, effectiveness prediction using machine learning assists in selecting the best candidates for clinical trials, potentially reducing the risk of costly failures. This approach enhances decision-making in the drug development process and contributes to the progression of personalized medicine strategies.
The Interplay Between AI and Genomic Data
Integrating artificial intelligence with genomic data holds transformative potential in healthcare. Innovations include analyzing vast genomic datasets and designing predictive models for early detection of genetic conditions.
Mining Genomic Data with AI
Mining genomic data using AI techniques enables detailed analysis of massive datasets that would be challenging for humans alone. AI algorithms, particularly machine learning, extract patterns and insights from vast genomic sequences. These patterns help in identifying genetic variations associated with specific diseases, enhancing diagnostic accuracy.
AI systems can efficiently handle the massive data generated by next-generation sequencing technologies. By integrating structured and unstructured data, AI facilitates comprehensive genomic data interpretation. These advancements expedite the discovery of genetic markers crucial for personalized medicine, paving the way for more targeted therapies and interventions.