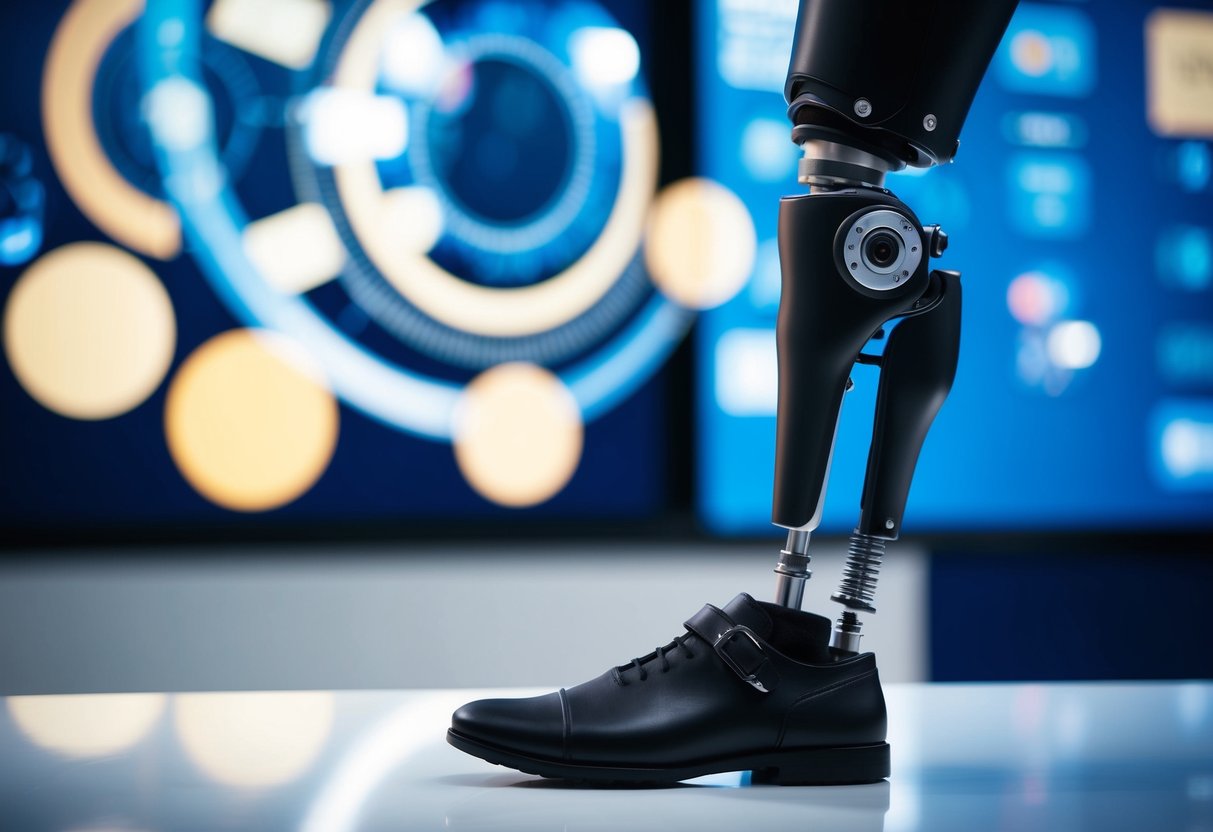
Role of Machine Learning Algorithms
Machine learning algorithms play a vital role in AI-enhanced prosthetics by enabling adaptive learning and improved functionality over time. These algorithms process the complex data received from neural interfaces, learning the user’s movement patterns and intentions. As the algorithms learn and adapt, the prosthetic can perform more efficient and natural movements. This adaptability is crucial for tasks that require fine motor skills and quick adjustments, such as picking up fragile objects. Researchers focus on developing algorithms that are capable of processing data in real-time, ensuring instant feedback and adjustment. Enhanced machine learning capabilities lead to more intuitive user experiences, providing prosthetics with the ability to predict and respond to user needs more effectively.
Biocompatibility Considerations
Biocompatibility is a fundamental consideration in the design of AI-enhanced prosthetics, ensuring that the materials used do not provoke an immune response when implanted in the body. The success of neural interfaces and electrodes hinges on their compatibility with human tissue. Researchers use biocompatible materials like titanium and certain polymers to create seamless integration. Long-term stability and minimizing risks, such as inflammation or infection, are central aspects of ongoing research. Effective biocompatibility also contributes to the user’s comfort and safety. By prioritizing these elements, developers ensure that prosthetics can be used reliably over extended periods, providing enduring support to individuals reliant on these technologies for mobility.
Brain-Computer Interfaces in Prosthetics
Brain-Computer Interfaces (BCIs) are revolutionizing prosthetics by enabling direct communication between the human brain and artificial limbs. This technology enhances mobility for individuals with amputations by offering greater control and natural movement of prosthetic devices.
Brain-Machine Interface Technology
Brain-Machine Interfaces (BMIs) connect the brain to external devices, thus bridging the gap between thought and action in prosthetics. This involves decoding neural signals that represent motor intentions. These decoded signals are converted into commands to actuate prosthetic limbs.
The development of high-resolution electrodes and advanced signal processing algorithms is crucial. Researchers focus on minimizing latency and enhancing signal accuracy for seamless integration. Key advancements include non-invasive brain signal acquisition methods, which reduce surgical risks and improve accessibility.
Neuroprosthesis Development
Neuroprostheses build on the principles of BCIs and BMIs to restore limb function. These devices interpret brain signals to mimic the natural movement of lost limbs, providing a means for users to perform complex tasks. Recent innovations incorporate feedback mechanisms, allowing users to receive sensory information such as touch or pressure.
Materials used in neuroprosthetic limbs are being engineered for flexibility and durability. Integration of sensory feedback into the neural network has been a significant focus, aiming to enhance user experience. Continued research explores ways to increase the adaptability of BMIs to individual neural architectures, further refining the efficacy of these remarkable devices in daily life.