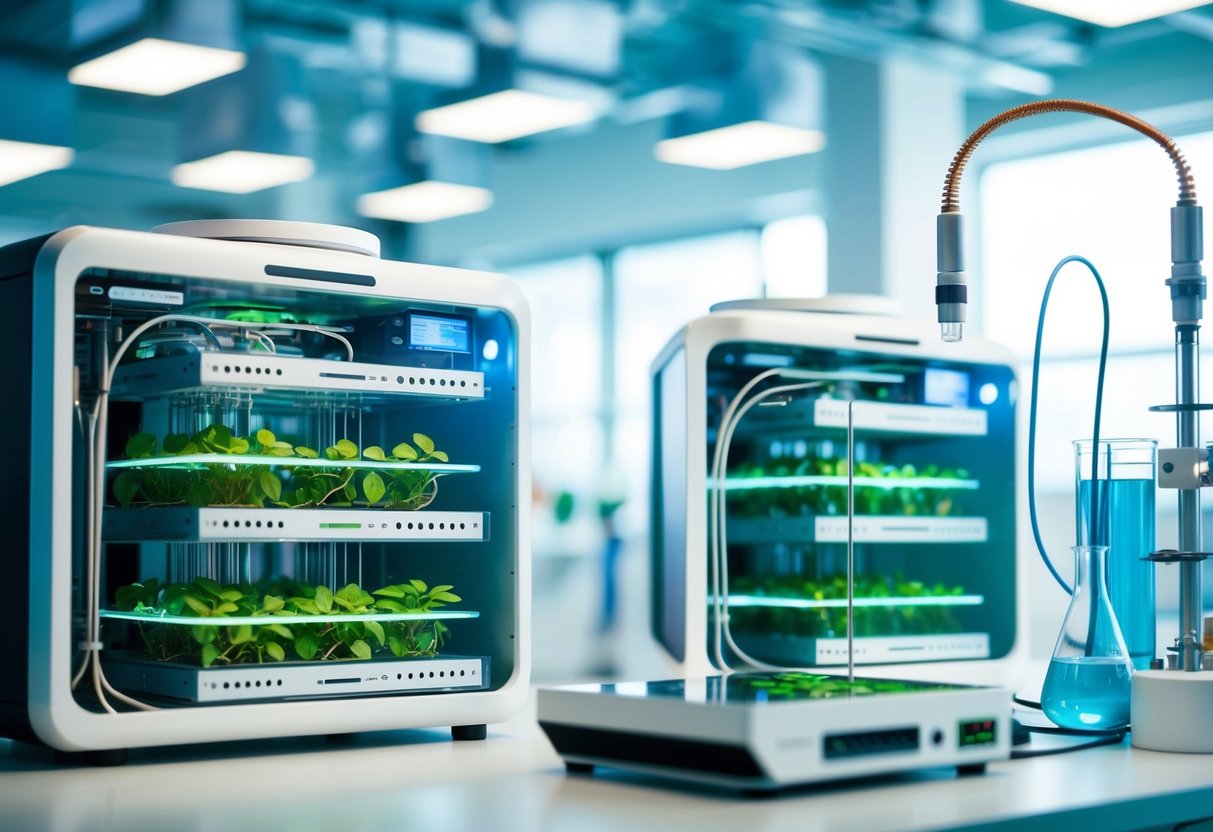
Logic Gates in Synthetic Biology
Logic gates are central elements within synthetic biology, allowing for the manipulation of genetic circuits. These biological counterparts to electronic logic gates—AND, OR, NOT—permit cells to compute logical operations. By using gene promoters and repressors, specific sequences produce desired cellular responses based on conditional inputs.
An example of a logic gate in synthetic biology is the AND gate, which requires multiple signals to trigger a response. When both conditions are met, a fluorescent protein may be expressed, providing a visible indicator. This functionality is crucial for advancements in biocomputing, enabling cellular systems to make decisions and execute complex tasks.
Research in logic gates within synthetic biology continues to evolve, aiming to increase reliability and minimize errors. Current developments focus on refining these systems to enhance computational capacity without compromising biological functions. This progress is essential for advancing biocomputers as practical and efficient alternatives to traditional silicon-based systems.
Organoids and Neuronal Networks
Exploring the frontier of biocomputing encompasses the use of organoids and neuronal networks. These advancements demonstrate potential in revolutionizing computational methods by harnessing the natural abilities of human brain cells.
Brain Organoids as Computational Models
Brain organoids, clusters of human brain cells cultivated in laboratories, serve as fascinating computational models. They mimic the architecture and functionality of a developing human brain to a degree, providing insights into neurological and computational phenomena. Researchers utilize these organoids to study complex brain activities, which may pave the way for innovative biocomputing technologies.
Organoid intelligence refers to the potential for these structures to perform computational tasks. This area of study investigates how organoids can process information in ways that traditional computational hardware cannot. It represents a pioneering approach that brings biological principles into the realm of computing. Their development may lead to novel computational frameworks that integrate biological and electronic systems.
Neurons: The Basic Units of Biocomputing
Neurons are integral to biocomputing as they transmit information through electrical and chemical signals. As the fundamental units of the nervous system, their structure and function inspire new computing paradigms. Neuronal networks, in which neurons communicate, offer models for parallel processing and learning.
In synthetic biocomputing, mimicking neuronal functions presents opportunities for creating highly efficient computational architectures. The study of neuron-based computing explores how these cells can be harnessed to perform calculations, offering potential advantages over conventional silicon-based processors. Understanding neuronal networks could lead to breakthroughs in data processing and machine learning, offering new insights into building computing systems.
Empowering Artificial Intelligence
Biocomputing is transforming the landscape of artificial intelligence by incorporating biological systems that enhance computational capabilities. It serves as a pivotal player in advancing AI technologies by leveraging unique properties of neuronal networks and organoid intelligence.
Biocomputing’s Role in Advancing AI
Biological systems introduce significant innovation in AI development. Biocomputing uses living cells to perform computations, making systems more adaptable and efficient. Traditional computing hardware faces limitations in processing capabilities and scalability; by contrast, biocomputing offers a solution by simulating processes similar to human thought.
This method incorporates neuronal networks, which are a step closer to mimicking the complexity of the human brain. These networks can process information in a decentralized manner, offering improvements in speed and accuracy. The adaptability of these biological elements means they can learn and evolve, breaking conventional barriers seen in electronic methods.