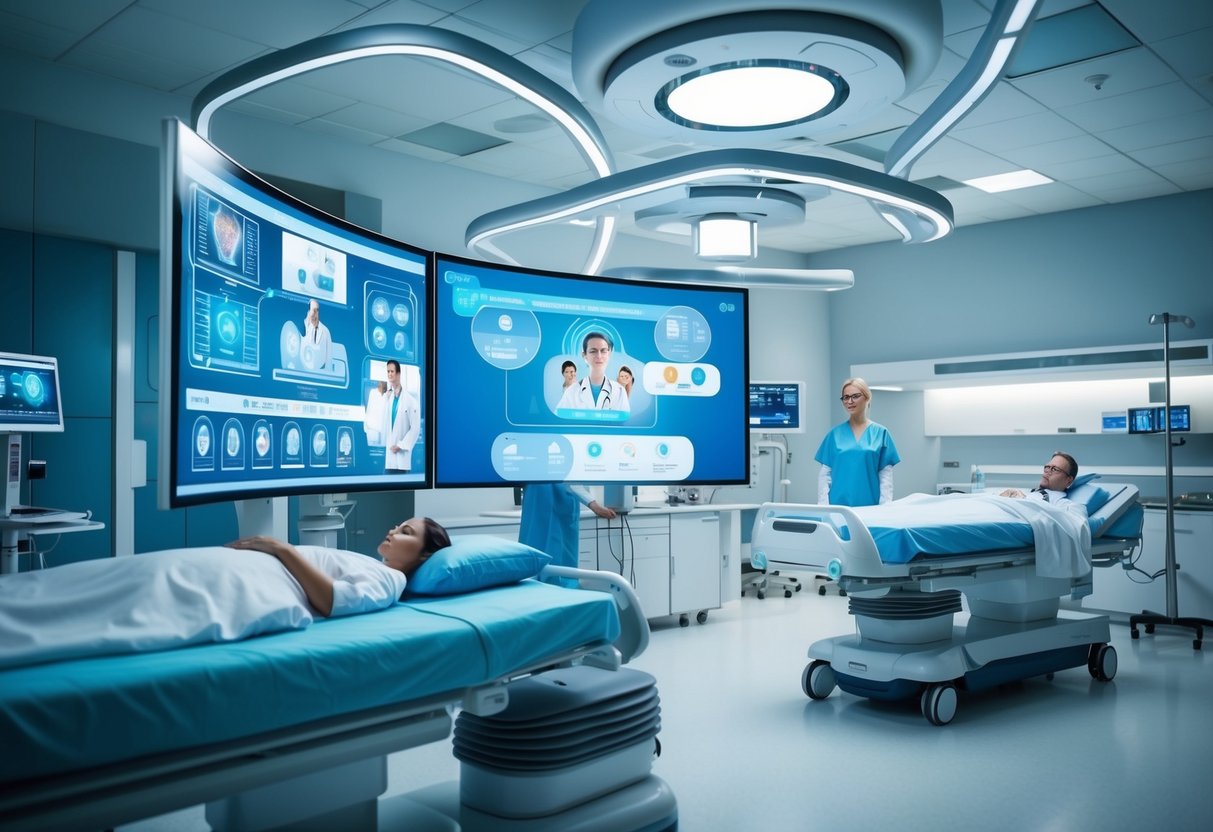
The landscape of healthcare is undergoing a transformation with the advent of AI-powered predictive tools, which are reshaping the way illnesses are anticipated and managed. By analyzing vast amounts of data, predictive analytics can foresee potential health issues before symptoms even arise. This capability allows for preemptive treatments, reducing complications and improving patient outcomes.
In proactive healthcare, AI’s role is pivotal in moving from a reactive to a preventive model. With these technologies, healthcare providers can offer insights that guide lifestyle changes and early interventions, potentially decreasing the incidence of chronic conditions. This shift signifies not only a step forward in patient care but also a reduction in healthcare costs by focusing on prevention rather than treatment.
The integration of AI in healthcare is enabling a closer alignment between patient lifestyle and medical advice, fostering more personalized care plans. These advances aim to give patients a more significant role in their health journey, facilitating informed decisions and encouraging healthier choices. The potential for AI to enhance these aspects of healthcare is profound, promising a more efficient and effective system.
Understanding AI in Healthcare
Artificial Intelligence has transformed healthcare by offering advanced capabilities in early detection, diagnosis, and patient management. This transformation is largely driven by the evolution of AI technologies and the integration of these into healthcare systems.
Evolution of AI Technologies
AI technologies have evolved rapidly, marked by significant advancements in machine learning and deep learning methodologies. From early rule-based systems to today’s sophisticated algorithms, AI now processes vast amounts of data for insightful analyses. In healthcare, this evolution enables automated pattern recognition in medical images and predictive analytics for patient outcomes. Increased computational power and more accessible big data have propelled these advances, enhancing the ability of AI systems to provide efficient decision support.
Key Concepts in AI and Healthcare
The application of AI in healthcare centers around several key concepts. Machine learning models are trained on extensive datasets to identify patterns and insights that would be impractical for humans to detect. AI aids in diagnostic accuracy and in tailoring personalized treatment plans. Decision support systems based on AI provide clinicians with data-driven insights that improve patient care outcomes. Digital transformation in healthcare is largely fueled by AI’s ability to integrate with existing systems, enhance operational efficiency, and reduce costs.
AI and Predictive Analytics
AI and predictive analytics are transforming the healthcare industry by enabling personalized treatments and improving prevention strategies. These technologies leverage data-driven techniques and precision tools to accurately predict health outcomes and assess risks.
Predictive Modeling Techniques
Predictive modeling in healthcare uses advanced algorithms to forecast potential health outcomes. Neural networks and other machine learning frameworks analyze complex datasets from clinical trials, patient history, and more. By identifying patterns and trends, these models help clinicians make data-driven decisions effectively. Predictive analytics can vastly change disease management by anticipating disease progression and optimizing treatment plans before conditions worsen. However, the risks of algorithmic bias remain a concern, requiring continuous evaluation and refinement to enhance precision and fairness.
Risk Assessment Tools
Risk assessment tools in predictive analytics are crucial for identifying patients at high risk of developing certain health conditions. These tools utilize data from various sources, including electronic health records, to create accurate risk profiles. Risk prediction instruments enable healthcare professionals to provide targeted preventive measures, reducing the incidence of severe illnesses. Despite their benefits, these tools can be susceptible to biases due to skewed or insufficient data. Continuous monitoring and adjustment ensure they remain reliable and equitable, fostering trust and effective patient care.