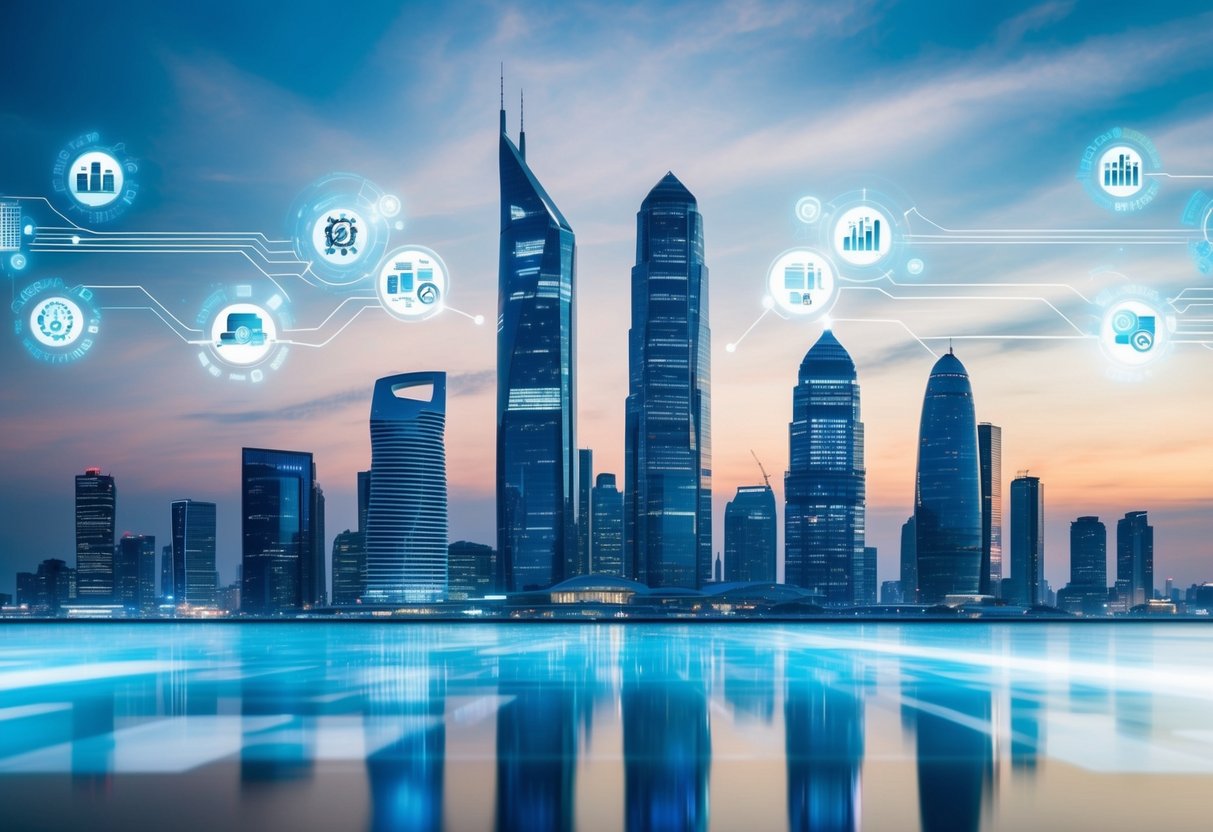
Machine Learning Algorithms for Credit Scoring
Machine learning algorithms are reshaping how credit scoring is approached by enhancing the accuracy of credit decisions and recognizing patterns in financial behavior. These advancements in analytical techniques are providing financial institutions with efficient and robust tools to evaluate creditworthiness.
Enhancing Credit Decision Accuracy
Machine learning algorithms play a crucial role in improving credit decision accuracy. They utilize vast datasets to analyze patterns and predict creditworthiness with greater precision. Unlike traditional models, these algorithms consider a broader range of variables. They can adapt to new data in real-time, enhancing the robustness of credit evaluations.
Credit scoring models using machine learning can incorporate alternative data sources, such as transaction history and even non-traditional financial indicators. This provides a more holistic view of an individual’s financial status. By continuously learning from new information, these algorithms can preemptively identify potential risks, offering lenders more reliable insights.
Pattern Recognition in Financial Behavior
Pattern recognition in financial behavior is key to transforming credit scoring methodologies. Machine learning algorithms excel at identifying trends and outliers, allowing for a deeper understanding of a borrower’s financial habits. They assess patterns such as spending behavior, savings habits, and income fluctuations to predict future credit behaviors.
Such tools can detect subtle shifts in financial activities, which may not be evident through conventional methods. This capability is essential in minimizing risks, as it helps in identifying potential defaults at an early stage. By recognizing these patterns, financial institutions can tailor their credit offerings, creating more personalized and risk-adjusted financial products.
Innovative Fraud Prevention Strategies
In the financial sector, innovative techniques are replacing older practices to enhance security and combat fraud. Technologies such as biometrics and encryption have become key elements in enhancing the defense mechanisms against evolving threats.
Biometrics and Encryption Methods
Biometrics introduces a layer of security by using unique biological traits like fingerprints or facial recognition. This method reduces the risk of fraud since these traits are difficult to replicate or steal. Financial institutions implement these systems, enabling secure and swift identity verification. On the encryption front, advanced algorithms protect sensitive data during storage and transmission, ensuring that information remains confidential even if intercepted by unauthorized individuals. Integration of these approaches offers a powerful shield against attackers looking to exploit security weaknesses.
Adapting to Evolving Threats
Adapting strategies to face evolving threats is crucial for effective fraud prevention. Financial institutions continuously analyze emerging patterns in cyber threats and develop countermeasures. Adopting artificial intelligence and machine learning helps in recognizing suspicious activities in real time. These technologies digest large datasets to offer insights into unusual behaviors, allowing companies to prevent fraud before it occurs. This proactive stance offers financial institutions an edge in protecting their clients’ assets and maintaining trust.
The Importance of Transparency in AI Systems
AI systems in financial services must prioritize transparency to build trust and enable seamless integration. Interpretability allows understanding of AI decisions, while continuous improvement helps refine processes via feedback loops.
Explainable AI and Model Interpretability
Explainable AI refers to models that make decisions transparent and understandable. In financial services, this is crucial as stakeholders need clarity on why a system makes specific predictions, such as identifying potential fraud or assessing credit risk. Interpretability helps in validating these models and ensuring they align with regulatory standards, reducing compliance risks and increasing confidence among users.
Interpretable models can detect biases or inaccuracies by examining the model’s decision pathways. This creates opportunities to address potential ethical or operational concerns. Decision-makers and customers benefit from greater confidence in AI-driven processes when they can understand how results are derived.
Continuous Improvement and Feedback Loops
Feedback loops facilitate ongoing refinement of AI systems by incorporating new data and insights. In financial services, this means constantly adapting to evolving market conditions, regulatory requirements, and emerging fraud tactics. Continuous improvement ensures that AI models remain effective and relevant over time.
Regular updates to algorithms help identify and mitigate overlooked risks or inaccuracies. By learning from past decisions and outcomes, AI systems can prevent similar errors in the future. Implementing continuous improvement strategies fosters resilience and agility, crucial for maintaining trust in AI applications within complex financial environments. Feedback loops play an essential role in refining models and enhancing their overall effectiveness.