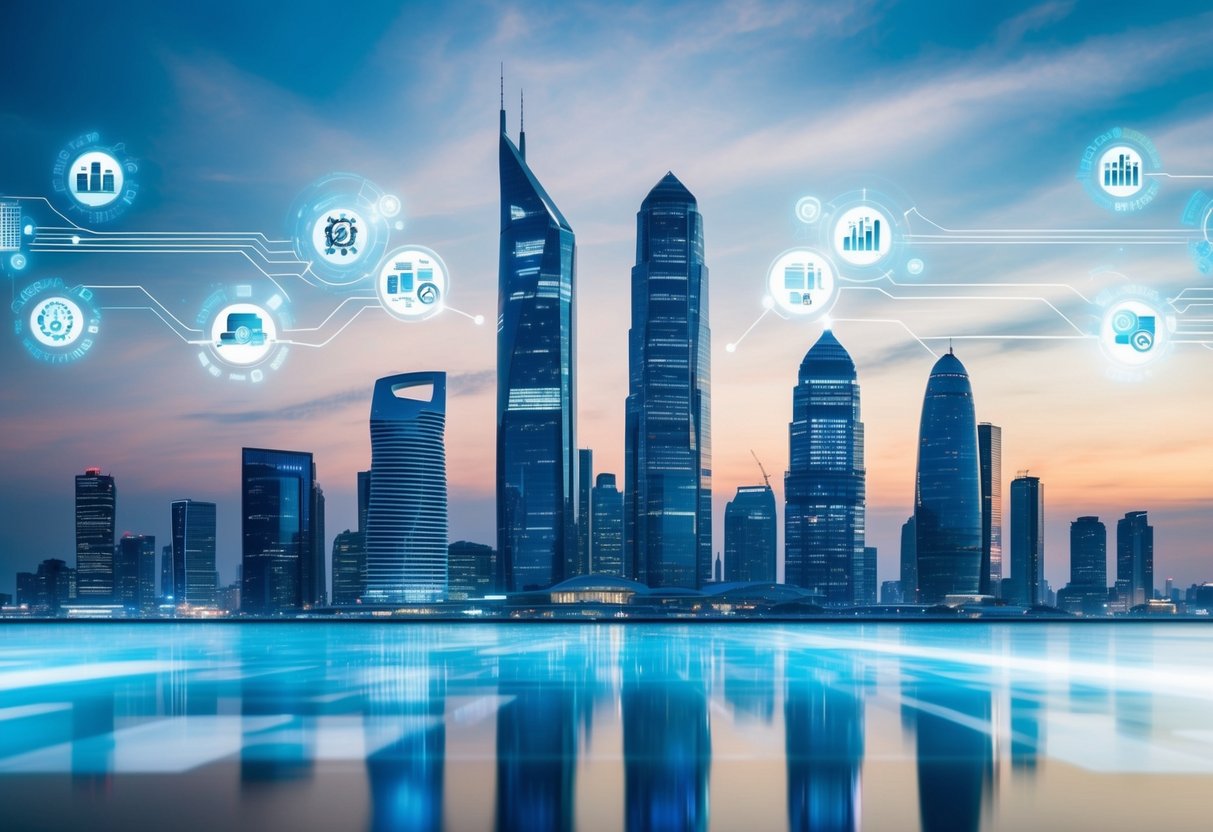
Compliance and Regulatory Considerations
AI’s integration into the financial services landscape demands a rigorous approach to compliance and regulatory adherence. Navigating complex laws and meeting sector-specific requirements are essential for maintaining trust and ethical standards.
Navigating Data Privacy Regulations
Data privacy laws are pivotal in AI deployment within financial services. Regulations such as GDPR and CCPA dictate how personal data should be collected, stored, and processed. Entities must ensure robust data protection mechanisms to prevent breaches. Implementing data anonymization and encryption strategies are fundamental in adhering to these regulations. Furthermore, organizations must focus on AI governance to align technological advancements with established data privacy norms, reducing risks connected with personal information misuse.
Meeting Financial Sector Compliance
The financial sector faces stringent compliance challenges, compounded by AI’s rapid advancement. Regulatory bodies establish frameworks to ensure AI’s application remains ethical and transparent. Companies must adapt processes and technologies to comply with standards such as AML requirements and KYC policies. Data governance becomes crucial in handling financial transactions. By establishing dedicated compliance teams, organizations can proactively address evolving regulations, ensuring AI solutions operate within legal boundaries.
Advanced Analytics and Data Management
In financial services, advanced analytics and data management enable firms to efficiently process large volumes of transaction data, facilitating the detection of potential risks and fraudulent activities. Through leveraging extensive data sets, financial institutions can gain insights into customer behaviors and preferences, while ensuring compliance with privacy regulations.
Leveraging Big Data in Finance
Big data plays a crucial role in transforming financial services by providing actionable insights from extensive datasets. Financial institutions utilize big data to analyze transaction records and customer profiles, helping to identify patterns that could indicate risk or fraud. They leverage these insights to tailor services to individual customer preferences and enhance overall decision-making processes.
Through sophisticated data analysis techniques, firms can predict potential market movements and customer behaviors. This anticipatory approach allows for more informed strategic planning and risk management. Data privacy and security remain paramount, as ensuring the protection of sensitive information is crucial for maintaining customer trust and regulatory compliance.
Real-Time Insights and Reporting
Real-time analysis enables financial institutions to respond swiftly to market changes and potential threats. By integrating real-time data into their operations, these institutions can offer up-to-date insights that drive effective reporting and decision-making. Real-time insights assist in monitoring and assessing transaction records, helping to quickly detect any anomalies.
Such timely access to information allows institutions to react proactively, minimizing potential risks and enhancing their fraud detection capabilities. Real-time reporting also facilitates improved transparency with stakeholders, ensuring that decisions are informed by the most current data available. This immediacy is vital for maintaining competitiveness and achieving optimal operational efficiency.
Technologies Behind AI in Financial Services
AI technologies are transforming financial services by enhancing risk management and fraud detection. These innovations utilize deep learning, natural language processing, and emerging technologies to bring precision and efficiency.
Deep Learning Applications
Deep Learning in financial services helps automate complex tasks like credit scoring and market prediction. Neural networks analyze vast datasets to identify trends and patterns. For risk management, these models can assess creditworthiness or detect fraudulent transactions in real-time.
Deep learning’s ability to handle unstructured data makes it invaluable for analyzing customer behavior. By learning from historical data, AI systems improve decision-making, ensuring more accurate predictions. Advanced prediction capabilities enhance investment strategies and minimize risks faced by financial institutions.
Natural Language Processing Use Cases
Natural Language Processing (NLP) enables the automation of tasks such as customer support, sentiment analysis, and compliance checks. In fraud detection, NLP analyzes transaction descriptions and communication logs to identify suspicious behavior. Chatbots powered by NLP provide real-time assistance to customers, enhancing user experience.
In financial document analysis, NLP extracts critical information from legal documents, contracts, and reports with accuracy and speed. This efficiency not only reduces processing times but also ensures compliance with regulations. Such capabilities streamline operations and boost overall productivity within the financial sector.
Emerging Technologies in Finance
Emerging technologies like Blockchain, Quantum Computing, and Generative AI are reshaping the financial landscape. Blockchain provides secure and transparent transaction records, reducing fraud risks. Smart contracts ensure transactions are executed only when certain conditions are met, enhancing security.
Quantum computing promises unparalleled computation speeds, potentially optimizing complex financial algorithms and decrypting data faster. Generative AI supports fraud detection by simulating potential security breaches, helping companies prepare better safeguards. These innovations together ensure a robust framework for financial operations, paving the way for future advancements.