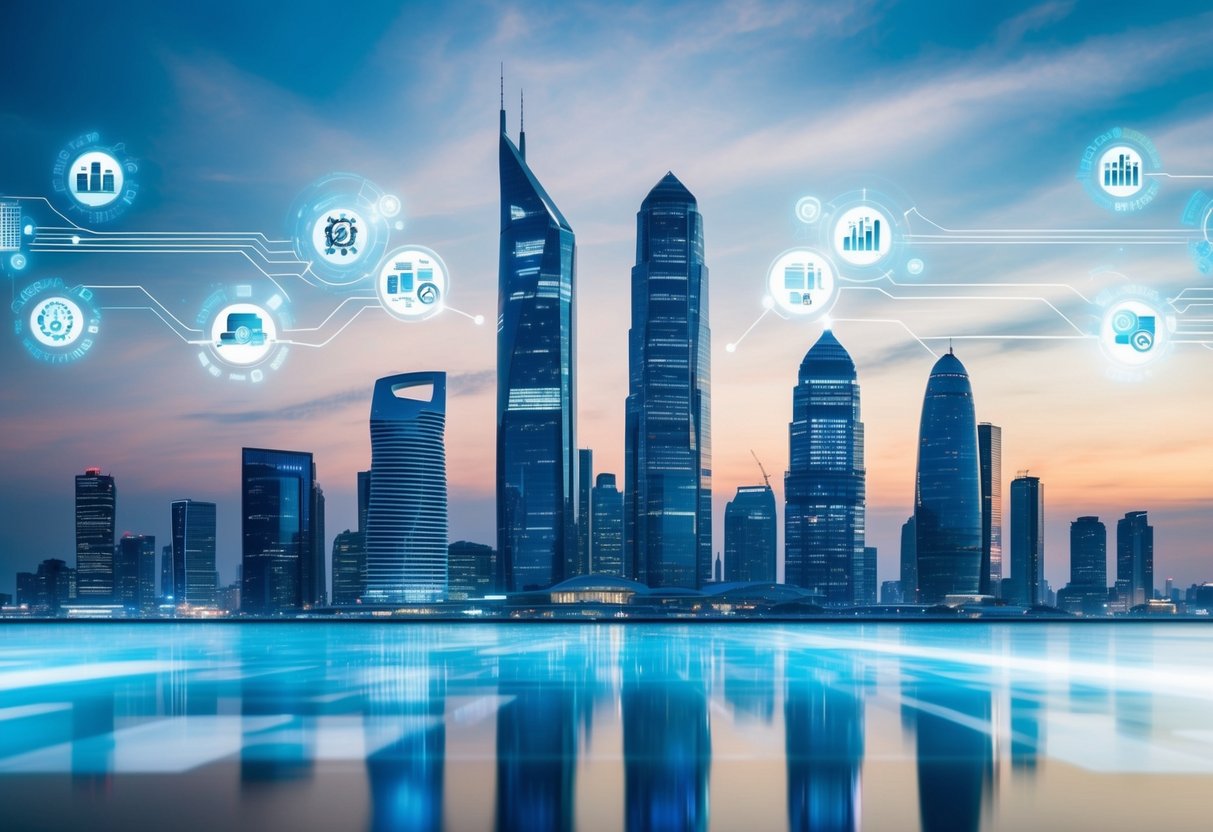
Predictive Analytics in Risk Evaluation
Predictive analytics utilizes AI to forecast potential risks and trends by examining historical data and current market conditions. These models generate actionable insights into potential disruptions, allowing companies to prepare strategies that mitigate risks before they manifest. This proactive approach is crucial in addressing emerging threats and maintains organizational resilience.
AI-driven predictive analytics can identify complex relationships in data, revealing subtle shifts in trends that may signal future risks. Such insights empower companies to fine-tune their risk management strategies, ensuring they are constantly aligned with the dynamic financial landscape and improving overall risk resilience.
Decision-Making Processes
Incorporating AI into decision-making processes empowers organizations to make data-driven choices swiftly and accurately. AI systems process vast amounts of data in real-time, providing timely recommendations based on current market conditions and historical outcomes. This enhances the agility and precision of financial entities when dealing with risk management decisions.
AI’s continuous learning capabilities ensure decision-making processes evolve, adapting to new threats and data patterns. Financial institutions leverage these capabilities to enhance their risk management frameworks, ultimately contributing to improved stability and strategic planning within the organization.
Automating Fraud Detection with Machine Learning
Machine learning is significantly enhancing fraud detection by identifying subtle patterns and minimizing false positives. These advancements help financial services detect fraudulent activities more effectively, ensuring tighter security and operational efficiency.
Anomaly Detection Systems
Anomaly detection systems play a crucial role in identifying unusual patterns in financial transactions. By leveraging machine learning algorithms, these systems can analyze vast volumes of data to pinpoint deviations from normal behavior. They recognize irregularities that may indicate fraudulent activities, such as sudden large transactions or atypical spending patterns. Incorporating these sophisticated techniques allows financial institutions to act swiftly, reducing potential losses and safeguarding customer accounts. Machine learning models continuously learn and adapt, improving their ability to detect new, emerging threats. They excel in environments where fraudulent patterns are constantly evolving, providing robust protection in the fight against financial crime.
Fraudulent Activities Recognition
Recognizing fraudulent activities requires identifying specific behaviors typical of fraudsters. Machine learning models are trained on historical data to detect known fraudulent signals, making it easier to catch those attempting to game the system. Techniques like supervised learning hone in on characteristics common to fraudulent endeavors, allowing systems to flag potential threats. This proactive approach not only deters would-be criminals but also protects consumers from financial harm. More accurate models mean financial institutions can develop more effective measures to combat fraud, ensuring higher trust and security for customers. Machine learning facilitates the dynamic recognition of new fraudulent patterns, enhancing a system’s ability to detect complex fraud schemes.
Reducing False Positives
Reducing false positives is vital for maintaining user experience and operational efficiency in fraud detection systems. When legitimate transactions are incorrectly flagged, it can lead to customer dissatisfaction and potential financial losses. Advances in machine learning help minimize these occurrences by refining the precision of detection algorithms. Models trained on vast datasets improve the discrimination between legitimate and suspicious activities, reducing unnecessary alerts. This balance ensures that authentic transactions proceed smoothly while only genuine threats trigger alerts. Continuous refinement of models supports financial institutions in maintaining high accuracy rates, preserving both security and customer trust. As algorithms evolve, the ability to reduce false positives improves, enhancing overall system performance.