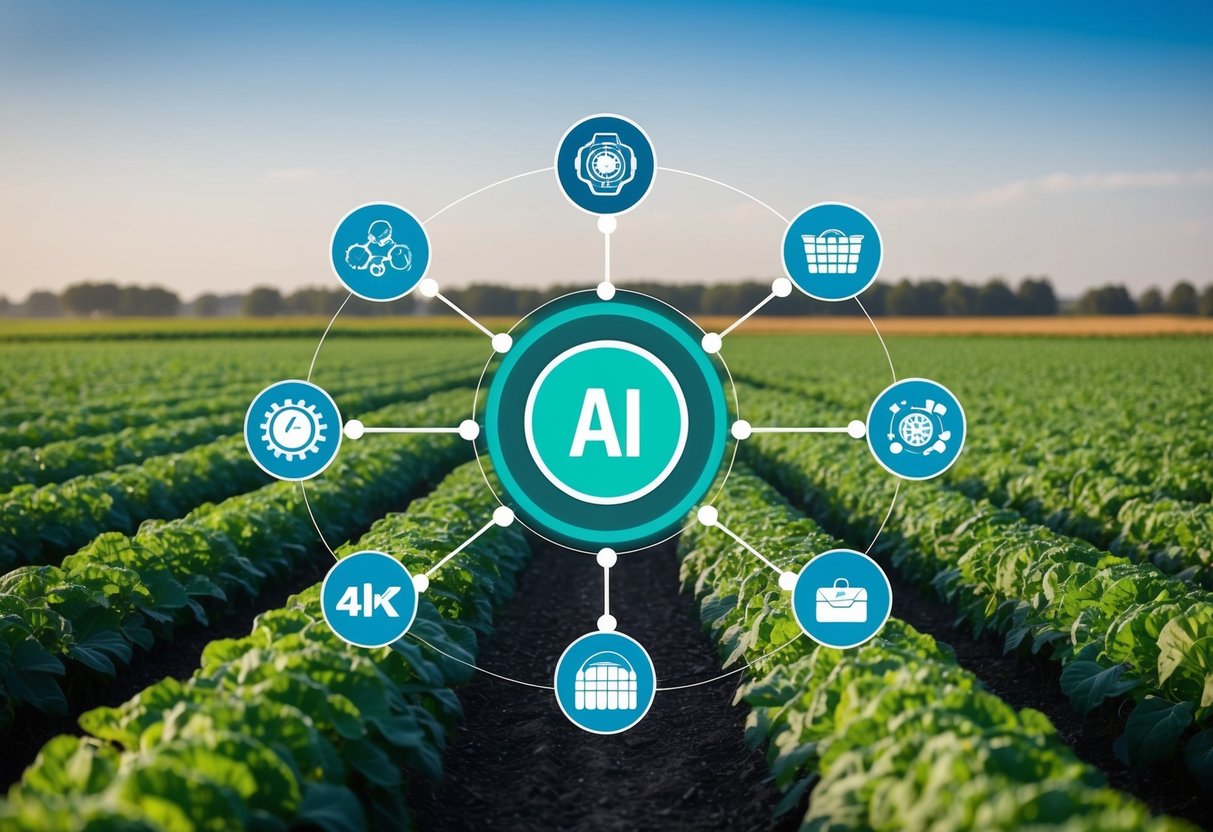
Ecological Monitoring and Climate Adaptation
Ecological monitoring with AI enables farmers to track and adapt to environmental variables with greater accuracy. By analyzing satellite imagery and data from IoT devices, AI can identify pest outbreaks, plant diseases, and other ecological threats in real-time.
This technology also facilitates the adaptation to climate change by forecasting weather patterns and suggesting timely interventions. Advanced AI algorithms help farmers make data-driven decisions that align with sustainability goals.
Predictive analytics forewarn of extreme weather events, giving agriculturalists time to implement protective measures. Thus, AI-driven ecological solutions not only address immediate challenges but also support long-term sustainable agricultural practices.
Integrating IoT for Enhanced Farming Operations
Incorporating IoT into agriculture optimizes farming by providing real-time monitoring and proactive risk assessment. This integration aims to create smarter farms, increase crop yields, and minimize environmental impact.
IoT Devices for Real-Time Monitoring
IoT devices like sensors and drones revolutionize modern farming. Sensors track soil moisture, temperature, and nutrient levels, offering farmers immediate insights. This data helps optimize water use and improve crop health. Drones equipped with cameras provide aerial views, identifying crop stress or pest infestations before they become severe problems.
Farmers gain better control over their operations through these devices. Remote access allows adjustments anytime, fostering efficient resource use. Connected machinery enhances planting, harvesting, and inventory management, improving productivity.
Real-time data means quicker decision-making and more efficient resource allocation, essential for sustainable farming practices.
Early Warning Systems and Risk Assessment
The integration of IoT in agriculture facilitates early warning systems. These systems alert farmers to potential risks such as pests, diseases, or extreme weather conditions. By analyzing data patterns, they predict potential threats to the crops, allowing timely interventions. This proactive approach mitigates risks and minimizes potential losses.
Advanced algorithms assess and categorize risks based on real-time and historical data. Customized notifications guide farmers in preventive actions, reducing reliance on reactive measures. These assessments are crucial for seasonal planning, ensuring flexibility in operations and enhancing supply chain resilience. As a result, this leads to a more stable and predictable food production cycle.
Advanced Learning Algorithms in Agricultural Health
Advanced learning algorithms are reshaping agricultural health by enhancing food safety and supply chain transparency. These technologies utilize both supervised and unsupervised learning techniques to predict disease outbreaks, monitor plant health, and analyze complex data patterns for improved decision-making.
Reinforcement and Supervised Learning Applications
Reinforcement and supervised learning are pivotal in agricultural health. Supervised learning uses labeled datasets to train algorithms for precise predictions in crop yield and pest management. By identifying correlations between environmental factors and plant health, it enhances agricultural productivity.
Reinforcement learning, meanwhile, involves algorithms learning through trial and error, much like a human would. This method aids in optimizing resource allocation and crop management decisions. Algorithms continuously improve as they process more data, leading to more efficient farming practices and better risk management. Together, these learning approaches enable farmers to adapt strategies proactively in response to real-time conditions, ensuring both productivity and sustainability.
Unsupervised Learning for Pattern Recognition
Unsupervised learning excels in identifying patterns and trends within datasets where explicit labeling isn’t available. It plays an essential role in agricultural health by uncovering hidden structures in soil composition, weather patterns, and crop diseases.
Through clustering and dimensionality reduction techniques, unsupervised learning helps in segmenting fields into zones requiring varying degrees of attention. This allows for targeted interventions, such as applying precise amounts of fertilizers or pesticides, minimizing waste and environmental impact. Unsupervised learning’s ability to recognize complex patterns without human intervention proves invaluable for comprehensive data analysis, ultimately supporting informed decision-making and reinforcing the efficiency of agricultural operations.