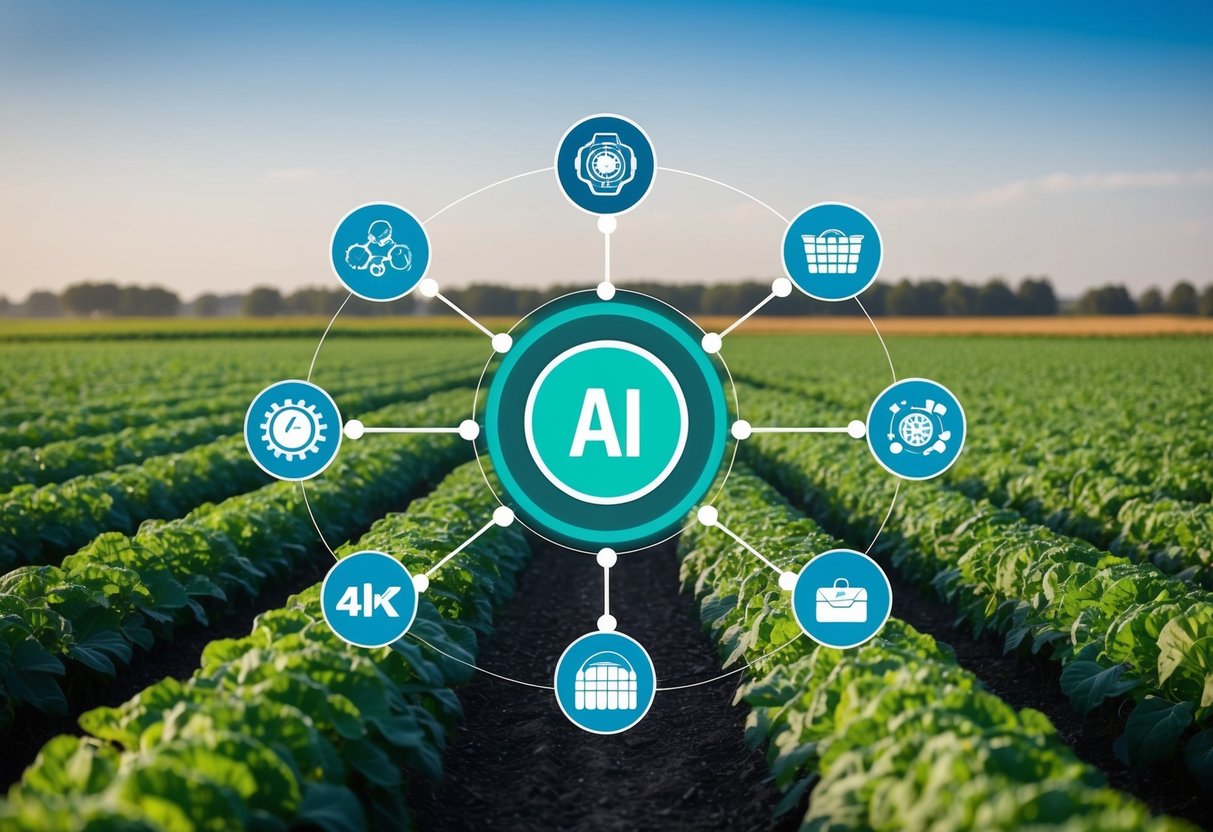
Spectroscopy and Advanced Imaging Technologies
Spectroscopy, combined with advanced imaging, enhances food quality assessment by identifying chemical composition and physical attributes. This approach uses non-destructive techniques to analyze the internal structure of food items, offering insights into freshness, ripeness, and potential contamination.
Advanced imaging technologies support the identification of ingredients and additives, ensuring that products comply with safety standards. These methods also enhance traceability in the supply chain, providing essential data for quality control.
Spectroscopy offers precision that other traditional methods might lack, strengthening food safety measures. This integration of imaging with spectroscopic analysis fosters transparency and trust in the agricultural sector.
Data-Driven Agriculture
In modern agriculture, data is a pivotal element in enhancing food safety and ensuring the transparency of supply chains. By examining large-scale datasets, farmers and agribusinesses can significantly boost productivity and optimize yields.
Leveraging Big Data in Crop Management
Big data plays a crucial role in transforming crop management practices. By collecting and analyzing vast amounts of information from various sources such as soil sensors, weather stations, and drones, farmers can gain insights into soil health, moisture levels, and pest infestations. Data analysis helps in making informed decisions about irrigation, planting schedules, and the application of fertilizers and pesticides. This level of precision increases agricultural productivity while minimizing resource wastage.
Moreover, integrating data from multiple channels allows for real-time monitoring and responsive actions to environmental changes. Advanced data platforms enable farmers to predict and mitigate risks associated with climatic conditions and market demands. As a result, the agricultural sector becomes more resilient and sustainable, equipped to face the challenges of a modern world.
Predictive Analytics for Yield Optimization
Predictive analytics is instrumental in optimizing crop yields. By utilizing historical data and machine learning models, farmers can forecast future crop performance based on variables like weather patterns and soil conditions. This anticipatory approach aids in aligning farming practices with optimal yield periods.
Data-fueled insights contribute to better resource allocation, ensuring that water, fertilizers, and seeds are used judiciously. Consequently, this improves the efficiency of farm operations and enhances crop quality. Through predictive analytics, potential issues such as disease outbreak or nutrient deficiency can be identified early, allowing for preventive measures. Embracing this technology empowers agricultural communities to achieve higher yields and meet the growing food demand globally.
Impact of AI on Sustainable Agricultural Practices
AI technologies are playing a crucial role in promoting sustainable agriculture by enhancing resource management and ecological adaptability. These innovations address challenges related to environmental sustainability and climate change.
Resource Optimization and Allocation
AI systems assist in the precise management of agricultural resources such as water, fertilizers, and pesticides. These technologies analyze data from various sources to provide insights that reduce unnecessary resource consumption.
For instance, AI-driven irrigation systems utilize sensors and weather data to determine optimal watering schedules, ensuring that water is used efficiently. Similarly, AI models can predict soil nutrient deficiencies, allowing farmers to apply the exact amount of fertilizers needed, minimizing waste and environmental impact. Through these methods, AI bolsters sustainable agriculture by optimizing input use, which further supports resource conservation efforts.