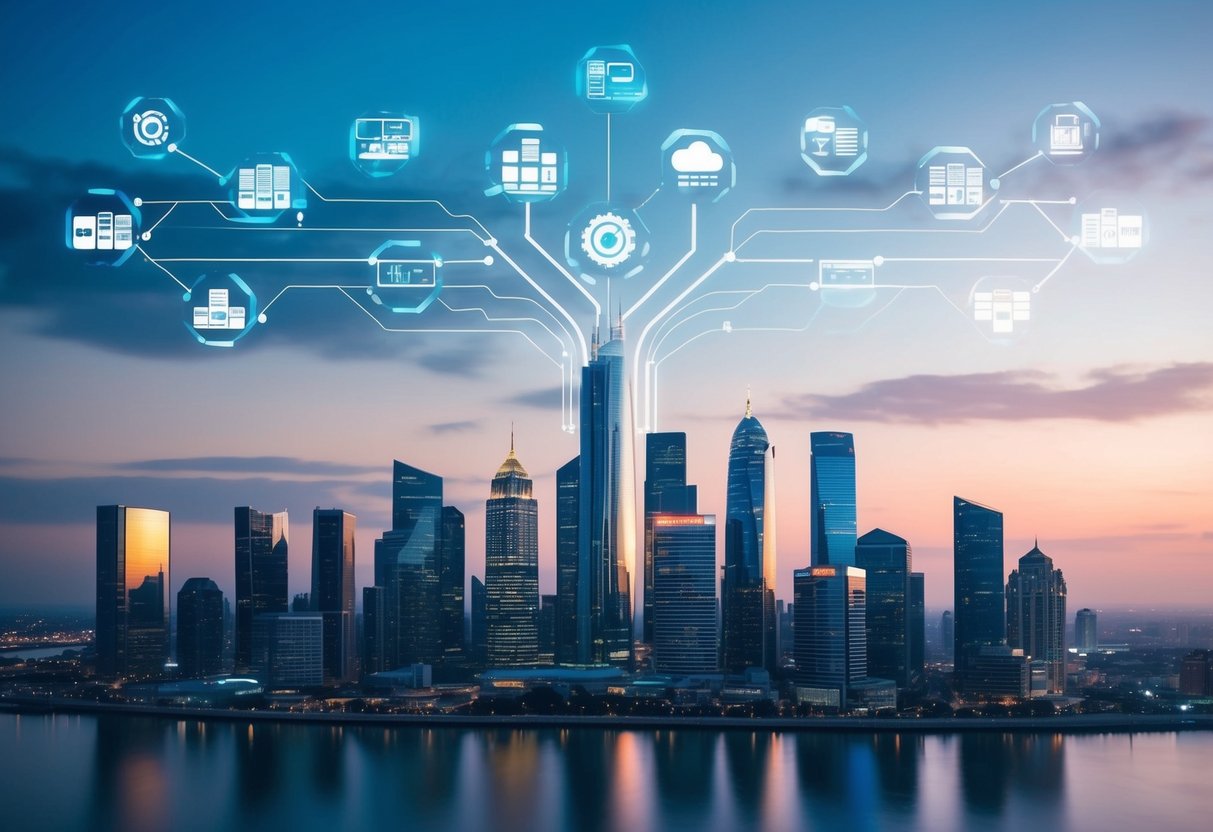
In the world of business, decision-making is increasingly being transformed by advances in predictive analytics within AI. Companies are leveraging algorithms to analyze vast amounts of data, uncovering patterns and insights that were previously hidden. This capability allows businesses to anticipate trends, optimize operations, and make informed decisions with a level of accuracy and speed previously unattainable.
Adopting predictive analytics in AI empowers businesses to move from reactive to proactive strategies. Whether in finance, healthcare, or retail, AI-driven insights enable organizations to identify opportunities, mitigate risks, and enhance customer experiences. By accurately forecasting consumer behavior or market dynamics, companies can allocate resources more effectively and tailor their approach to meet evolving demands.
The impact of predictive analytics on business is profound, influencing every aspect from strategic planning to day-to-day operations. As AI technology continues to advance, its role in shaping business decision-making will only grow more significant. Embracing this tool can be the key to staying competitive and achieving long-term success.
The Fundamentals of Predictive Analytics
Predictive analytics leverages AI and statistical algorithms to forecast future trends based on historical data. By understanding its basic principles and historical development, businesses can make informed decisions.
Understanding Predictive Analytics
Predictive analytics involves analyzing historical data to predict future outcomes. It employs a range of techniques, including statistical modeling and machine learning. These methodologies analyze patterns and relationships within data, enabling predictions about trends, customer behaviors, and potential risks.
The integration of AI enhances predictive capabilities by automating complex calculations and continuously improving prediction accuracy. Companies use these insights for strategic planning, inventory management, and improving customer satisfaction. As the data landscape continues to grow, refinement in predictive methods becomes paramount for staying competitive.
History and Evolution of Predictive Analytics
Predictive analytics has evolved significantly, originating from traditional statistics and decision sciences. Early applications relied on simple statistical methods. With the advent of computational power, sophisticated algorithms emerged, enhancing the scope and accuracy of predictions.
The integration of AI marked a significant shift, making real-time data analysis feasible. As technology advanced, predictive analytics expanded into various sectors like finance, healthcare, and marketing. This evolution reflects a continual push for precision and scalability, driven by an increasing volume of data and the need for rapid, data-driven decisions.
Predictive Analytics and Artificial Intelligence Integration
Integrating Artificial Intelligence (AI) with predictive analytics is transforming how data is leveraged for business decisions. Machine learning and deep learning augment traditional models, offering improved accuracy and efficiency.
Role of AI in Predictive Analytics
AI enhances predictive analytics by processing large datasets quickly and providing insights with precision. Machine learning, a subset of AI, enables systems to learn from data patterns without human intervention. This capability allows businesses to forecast trends and consumer behavior effectively.
Neural networks, including deep learning models, are particularly impactful. These systems can identify intricate patterns and relationships within data. By leveraging neural networks, organizations can improve decision-making processes and anticipate future outcomes with greater accuracy.
AI Algorithms for Predictive Modeling
AI algorithms form the backbone of predictive modeling. Techniques like regression, classification, and clustering are employed to analyze data and generate predictions. Decision trees and random forests are popular choices due to their intuitive nature and interpretability.
Deep learning models, such as convolutional and recurrent neural networks, offer advanced solutions for complex datasets. These models excel in tasks requiring high-level abstraction, making them essential tools for analyzing unstructured data like images, text, and time-series information.
The integration of these algorithms allows predictive models to evolve continually, refining their accuracy and providing businesses with actionable insights tailored to their specific needs.